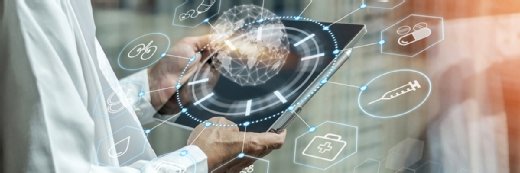
Chinnapong - stock.adobe.com
Data Analysis Model to Help Reveal Potential Infertility Treatments
Researchers studied polycystic ovary syndrome using a data analysis model to develop potential infertility treatment.
A data analysis model created by University of Minnesota researchers could identify potential treatments for women facing infertility issues.
Researchers examined data from 1,376 women with polycystic ovary syndrome (PCOS). While the condition is known to cause infertility, little data has indicated why.
According to the forthcoming study in the Journal of the American Statistical Association, “It has been a long-held hypothesis among the clinicians that some of the important variables for early pregnancy outcomes may continue their influence on live birth. To consider this possibility, we develop an l0l0-norm based regularization method in favor of variables that have been identified from an earlier stage.”
In PCOS, studies, the three stages of ovulation, pregnancy, and live birth are typically analyzed separately. However, fewer women progress to each stage, and only a small percentage reach live birth. Despite the challenge, the research team developed a series of algorithms and revealed effects present across all three stages.
“Our model bridges the three stages to look at the whole pregnancy process to see how different factors, like treatment or alcohol use, may affect the chance of a live birth,” Carlson School of Management Assistant Professor Xuan Bi said in a press release.
“The analysis shows how doctors could better identify a treatment at the start of the ovulation stage based on those factors.”
The data analytics model highlighted novel infertility treatment recommendations that improve the chances of a live birth. For instance, clomiphene citrate was more effective in older women. Additionally, the team also confirmed existing clinical research, such as the negative impact of smoking during pregnancy.
Bi said while the treatment recommendations should go through further clinical trials and FDA approval, the team’s findings look promising.
According to researchers, the new method could be applied to other clinical research of subsequent processes that struggle with similar diminishing data issues. In addition, Bi said the model could eventually be developed in a medical artificial intelligence tool used by doctors as a second option.
“This could be a step toward a potential software in a doctor’s office, where a patient could enter risk factors and other information to help augment a doctor’s decision for treatment,” said Bi.
Bi’s collaborators on the paper included Assistant Professor Long Feng at the City University of Hong Kong, Assistant Professor Cai Li at the St. Jude Children’s Research Hospital, and Professor Heping Zhang of the Yale School of Public Health.