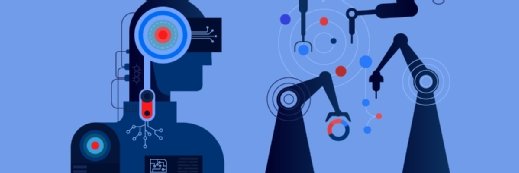
Getty Images
AI Imaging Techniques, Clinical Data Improve Predictive Models
Researchers use artificial intelligence and clinical data to predict a patient’s risk for heart attacks.
With the assistance of artificial intelligence imaging techniques and clinical data, physicians can improve predictive models indicating a patient’s risk for heart attacks.
When analyzed together in an artificial intelligence model, researchers found that coronary 18F-NaF uptake on PET and quantitative coronary plaque characteristics on CT angiography were complementary and robust predictors of heart attack risk in patients with established coronary artery disease.
Working together, the two techniques could provide heart attack risk prediction superior to that of clinical data alone.
In clinical practice, predicting a heart attack is difficult. The predicted likelihood of a heart attack is commonly based on cardiovascular risk factors and scores, especially in those with suspected coronary artery disease. However, cardiovascular risk factors and scores don’t always provide the complete picture in individuals with diagnosed coronary artery disease.
“Recently, advanced imaging techniques have demonstrated considerable promise in determining which coronary artery disease patients are most at risk for a heart attack. These techniques include 18F-sodium fluoride (18F-NaF) PET, which assesses disease activity in the coronary arteries, and CT angiography, which provides a quantitative plaque analysis,” Director of Innovation in Imaging at Cedars-Sinai Medical Center Piotr J. Slomka, PhD, FACC, FASNC, FCCPM, said in a press release.
“Our goal in the study was to investigate whether the information provided by 18F-NaF PET and CT angiography is complementary and could improve prediction of heart attacks with the use of artificial intelligence techniques.”
Around 300 patients with established coronary atherosclerosis participated in the study. Every patient underwent a baseline clinical assessment with an evaluation of their cardiovascular risk factor profile.
“All patients received hybrid coronary 18F-NaF PET and contrast CT coronary angiography. Machine learning — a type of artificial intelligence — was used to calculate a joint score for heart attack risk by incorporating key variables from the clinical assessment, 18F-NaF PET findings and quantitative CT variables,” the press release stated.
The machine learning model indicated a substantial improvement in predicting a heart attack over clinical data alone. According to researchers, this approach highlights that 8F-NaF PET and CT angiography are complementary and additive, with the combination of both leading to the strongest predictive outcomes.
“18F-NaF PET combined with anatomical imaging provided by CT angiography has the potential to enable precision medicine by guiding the use of advanced therapeutic interventions,” noted Slomka.
“Our study supports the use of artificial intelligence methods for integrating multimodality imaging and clinical data for robust prediction of heart attacks.”