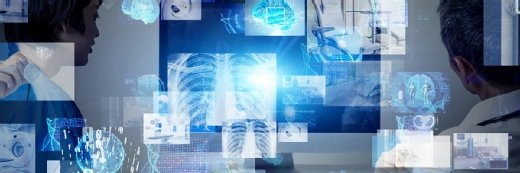
metamorworks/istock via Getty Im
Using Machine Learning For Personalized Breast Cancer Screenings
Researchers develop risk-based guidelines for personalized breast cancer screenings using machine learning.
Researchers from MIT’s Computer Science and Artificial Intelligence Laboratory (CSAIL) and Jameel Clinic for Machine Learning and Health studied the use of machine learning to personalize breast cancer screenings.
Currently, mammograms are the most widely used method for breast cancer screening. However, the method comes with a swirl of controversy regarding when and how often a mammogram should be administered.
While some advocates argue that women between the ages of 60- 69 who receive a mammogram have a 33 percent lower risk of death than those not receiving mammograms, others argue mammograms produce high costs and false positives.
According to a meta-analysis of three randomized trials, mammograms have a 19 percent over-diagnosis rate.
“Even with some saved lives, and some overtreatment and overscreening, current guidelines are still a catch-all: Women aged 45 to 54 should get mammograms every year. While personalized screening has long been thought of as the answer, tools that can leverage the troves of data to do this lag behind,” the press release stated.
To improve patient care, researchers investigated whether machine learning could be used to provide personalized screenings. Out of this came a technology for creating risk-based screening guidelines called Tempo.
Using an AI-based risk model, Tempo can recommend a specific date for a patient to return for a mammogram. The same Tempo policy can be adapted to a wide range of screening preferences, which would allow clinicians to pick their desired early-detection-to-screening-cost-trade-off, without training new policies.
The model was trained using a large screening mammography dataset from Massachusetts General Hospital (MGH). Using the team’s previously developed risk assessment algorithm Mirai, Tempo obtained better early detection than annual screenings while also requiring 25 percent fewer mammograms overall.
“By tailoring the screening to the patient’s individual risk, we can improve patient outcomes, reduce overtreatment, and eliminate health disparities,” lead researcher and PhD student in electrical engineering and computer science, Adam Yala, said in a press release.
“Given the massive scale of breast cancer screening, with tens of millions of women getting mammograms every year, improvements to our guidelines are immensely important.”
According to the press release, early AI use in medicine dates back to the 1960s. Deep medicine has significantly advanced drug diagnostics, predictive medicine, and patient care sixty years later.
“Current guidelines divide the population into a few large groups, like younger or older than 55, and recommend the same screening frequency to all the members of a cohort. The development of AI-based risk models that operate over raw patient data give us an opportunity to transform screening, giving more frequent screens to those who need it and sparing the rest,” Yala said.
“A key aspect of these models is that their predictions can evolve over time as a patient’s raw data changes, suggesting that screening policies need to be attuned to changes in risk and be optimized over long periods of patient data.”
Using reinforcement learning, Tempo developed a “policy” that predicts follow-up recommendations for each patient. The team first trained a neural network to predict future risk assessments. The model then estimates the patient’s risk to create risk-based screening policies.
The team then trained the policy to maximize the reward to the retrospective training set. The model then generates a recommendation for when the patient should return for their next screening, promoting early detection of chronic disease.
“Our framework is flexible and can be readily utilized for other diseases, other forms of risk models, and other definitions of early detection benefit or screening cost. We expect the utility of Tempo to continue to improve as risk models and outcome metrics are further refined,” Yala said.
“We’re excited to work with hospital partners to prospectively study this technology and help us further improve personalized cancer screening.”