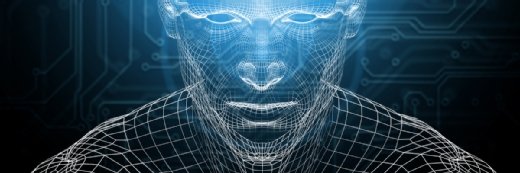
Getty Images
Artificial Intelligence Can Help Free Up Anesthesiologists' Time in OR
Researchers found that using artificial intelligence to administer drugs during surgery allows anesthesiologists to carry out their other responsibilities in the operating room.
Artificial intelligence systems may be able to assist anesthesiologists in the operating room, according to MIT and Massachusetts General Hospital (MGH) researchers.
In a special edition of Artificial Intelligence in Medicine, a team of neuroscientists, engineers, and physicians demonstrated how a machine-learning algorithm could continuously automate the dosing of the anesthetic drug propofol.
With, the algorithm outperformed traditional methods in patient simulations. It also closely matched the performance of real anesthesiologists with regard to maintaining unconsciousness using recorded data from nine actual surgeries.
The algorithm’s advances increase the possibility of using computers to maintain patient unconsciousness, freeing up anesthesiologists for all the other responsibilities they have in the operating room, such as making sure patients remain immobile, experience no pain, remain physiologically stable, and receive sufficient oxygen.
“One can think of our goal as being analogous to an airplane’s auto-pilot where the captain is always in the cockpit paying attention,” the study’s corresponding author Gabe Schamberg said in a press release. “Anesthesiologists have to simultaneously monitor numerous aspects of a patient’s physiological state, and so it makes sense to automate those aspects of patient care that we understand well.”
Additionally, the algorithm can assist in optimizing drug dosing to improve patient care.
“Algorithms such as this one allow anesthesiologists to maintain more careful, near-continuous vigilance over the patient during general anesthesia,” senior author Emery N. Brown, a neuroscientist at the Picower Institute for Learning and Memory and Institute for Medical Engineering and Science at MIT and an anesthesiologist at MGH, said in the press release.
The researchers created a machine-learning approach that would not only learn how to dose propofol but also how to optimize the amount of the drug administered.
According to the press release, the research team accomplished this by endowing the software with two related neural networks: an “actor” to decide how much of the drug to dose at every given moment, and a “critic” whose responsible for helping the actor behave in a manner that maximizes “rewards” specified by the programmer.
“For instance, the researchers experimented with training the algorithm using three different rewards: one that penalized only overdosing, one that questioned providing any dose, and one that imposed no penalties,” the release stated.
In every case, the team trained the algorithm with patient simulations that employed advanced models of both pharmacokinetics, how quickly propofol doses reach the relevant regions of the brain after doses are administered, and pharmacodynamics, or how the drug alters consciousness when it reaches its destination.
Simulated brain waves measured patient unconsciousness levels. By running hundreds of simulation rounds, both the actor and the critic could learn how to perform their roles for various patients.
After training and testing the algorithm with simulations, the researchers tested the “dose penalty” version — in which the critic questioned every dose the actor gave, constantly instructing the actor to keep dosing to a necessary minimum to maintain unconsciousness — by feeding it patient-consciousness data from actual cases in the operating room.
The research team found that the “dose penalty” was the most effective reward system.
But the testing also demonstrated both the strengths and limits of the algorithm.
During most of the tests, the algorithm’s dosing choices closely aligned those of anesthesiologists. Further, the algorithm adjusted dosing as frequently as every five seconds while anesthesiologists typically did so every 20-30 minutes.
However, researchers noted that the software does not know of its own accord when surgery is over.
One of the major challenges of any AI system is judging whether the information the technology is being fed is unbiased and accurate. Moving forward, the research team plans to improve their data sources and the quality of patient monitoring.