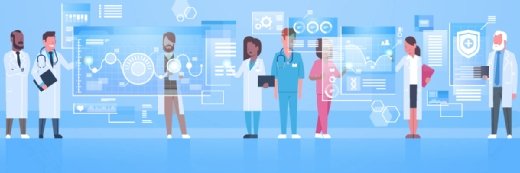
mast3r - stock.adobe.com
Florida Health System Uses AI to Predict Hepatitis C Treatment Outcomes
Using data from a national hepatitis C registry, researchers leveraged artificial intelligence to train algorithms to identify 41 risk factors impacting treatment success.
University of Florida Health researchers have developed algorithms using artificial intelligence to accurately predict hepatitis C treatment outcomes.
The algorithms use machine learning to produce treatment-failure predictions that are more accurate than current statistical models, according to a new study.
Researchers stated that successful hepatitis C treatment is critical to both patients and payers. The hepatitis C virus causes inflammation, leading to severe liver damage and high healthcare costs.
“No one wants the treatment to fail, least of all the patient,” the study’s lead researcher and UF College of Pharmacy Associate Professor Haesuk Park, PhD, said in a press release. “We wanted to find a better way.”
The research team developed four machine-learning algorithms to predict direct-acting antiviral treatment failure among hepatitis C patients. Almost 5,000 patient samples from a national hepatitis C registry were used to train the algorithms.
Another 1,631 patient samples were used to separately validate the algorithms’ effectiveness. Patients in the study were treated with one of seven oral hepatitis C medications from February 2014 to 2018.
According to Park, the algorithms were developed using 41 factors that put patients at risk for treatment failure. After developing and testing four common machine-learning algorithms, a technique known as gradient boosting machine or GBM was found to be the most accurate.
GBM stood out for its ability to identify the patients at the highest risk of treatment failure and place them in risk groups. The research team explained that identifying risk groups could be highly beneficial to physicians.
Machine learning also allowed researchers to distinguish specific conditions associated with hepatitis C treatment failure, including tobacco and alcohol use, diabetes, high blood pressure, and certain non-prescription medications used to treat acid reflux disease. Factors such as smoking, drinking, and taking certain acid reflux pills are modifiable to improve patients’ success with hepatitis C treatment.
While direct-acting antiviral treatments have initial cure rates of 95 percent or greater, even a tiny percentage of failures can have a significant impact. According to the World Health Organization, there are an estimated 58 million global cases of chronic hepatitis C, with 1.5 million new infections per year.
With machine learning, physicians are provided strategic support in making critical medical decisions.
While further refinement and work are necessary to understand how the team’s findings can be incorporated into clinical practice to improve patient outcomes, researchers see the machine learning model becoming part of electronic health records to generate alerts for high-risk patients before hepatitis C treatments are started.
“This is the first AI model developed to predict direct-acting antiviral treatment failure,” Park said. “This is a good foundation for future research.”