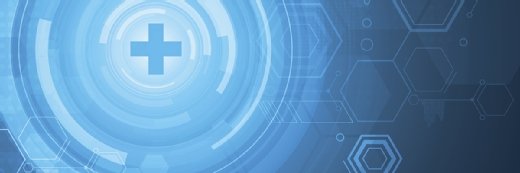
traffic_analyzer/DigitalVision V
New MIT Technique Aims to Boost Fairness Within Machine-Learning Models
A new study shows that reducing bias within machine-learning models is possible with a new technique that can help accurately map similarities and differences in data.
Recognizing the harm that unbalanced data can bring to the creation of machine-learning models, a study shows how Massachusetts Institute of Technology (MIT) researchers attempted to increase fairness in these models through a new technique, called Partial Attribute Decorrelation.
Researchers from MIT acknowledged that many machine-learning models were created using skewed data, causing them to produce uneven results.
Though the resultsproduced by the model are irreversible, researchers believe that adjusting the model itself can assist in creating better outcomes, despite being developed based on skewed data.
“In machine learning, it is common to blame the data for bias in models. But we don’t always have balanced data. So, we need to come up with methods that actually fix the problem with imbalanced data,” said lead author Natalie Dullerud, a graduate student in the Healthy ML Group of the Computer Science and Artificial Intelligence Laboratory (CSAIL) at MIT, in the press release.
Using the deep metric learning technique, researchers trained the neural network to recognize photos that are similar and different with regard to facial recognition and skin tone.
During this process, researchers uncovered more information about why the previous models produced unfair results.
They found that two people with a lighter skin tone were more likely to be differentiated than two people with a darker skin tone. Also, if models are trained taking into consideration the majority group instead of the minority group, it would cause bias.
To eliminate this issue, researchers created the Partial Attribute Decorrelation (PARADE). The goal of using this resource is to train models to recognize features it usually wouldn’t, making it easy to distinguish various faces.
They tested the method on two tasks, facial recognition and classifying bird species. The method reduced performance gaps caused by bias, researchers found.
The research will be presented at the International Conference on Learning Representations.
Looking ahead, Dullerud and other researchers are attempting to figure out how to create unbiased machine-learning models rather than relying on fixing them.
“How do you properly audit fairness? That is an open question right now. How can you tell that a model is going to be fair, or that it is only going to be fair in certain situations, and what are those situations? Those are questions I am really interested in moving forward,” said Dullerud in the press release.
Increasingly providers are turning to AI to improve clinical care. For example, Intermountain Healthcare created a Data Science and Artificial Intelligence Center of Excellence, which includes experts from the computer science, behavioral science, and data analytics arenas, to help it uphold ethical standards while developing and using AI.
The importance of accuracy when implementing machine learning is also critical, especially when it is responsible for various tasks within the operating room. For example, past research shows how machine learning can monitor patients’ brain activity during anesthesia. Although this is highly convenient, any errors could result in severe malfunctions during surgical procedures.