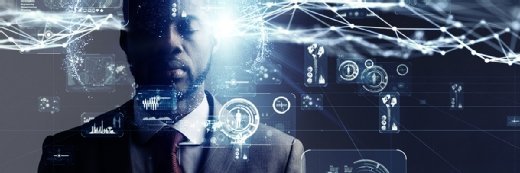
Getty Images
Computer Vision Model Improved Quality of Head MRIs
A new study shows how UK-based researchers created a computer vision model to speed up and improve the quality of head magnetic resonance imaging exams.
Following the increased demand for magnetic resonance imaging (MRI) and the shortage of radiologists in recent years, a study published in Medical Image Analysis shows how creating a computer vision model can improve MRI production quality and reduce the time taken to report abnormal head exams.
Computer vision models are designed to derive meaningful information from digital images and videos, according to IBM.
The study included 81,936 MRIs performed on adult brains at King’s College Hospital NHS Foundation Trust and Guy’s and St Thomas’ NHS Foundation Trust.
The text of each of these MRIs contained five to 10 sentences of image interpretation, taken from the Computerized Radiology Information System (CRIS).
Researchers believe that the most robust and most applicable computer vision models include various factors such as sensitivity to any abnormality, the ability to tell what an age-appropriate response is, the ability to capture slice-wise and voxel-wise views of images, flexibility in working with various types of patients, and expertise with scans taking place in routine clinical examinations.
Intending to create a model that can be as applicable as possible, researchers noted that axial T2-weighted scans occurred in more than 90 percent of MRIs.
With a focus on these types of scans, researchers began developing models. The development process consisted of various steps, including dataset labeling, image pre-processing, model interpretability, experiments, and simulation study. The models were trained at scale using a Transformer-based neuroradiology report classifier.
In a simulation, the best model developed by the researchers was able to assist in the production of faster and more accurate MRIs, reducing the mean reporting time for abnormal examinations from 28 days to 14 days and from 9 days to 5 days at the two hospital networks, the study shows.
Following the identification of the highest performing model, researchers detailed the various strengths of the study.
These strengths included proving the reliability of a neuroradiology report, the benefits of collecting data from two hospitals, and the subjectivity of the models dedicated to axial T2-weighted and axial diffusion-weighted scans. Slice-wise and voxel-wise visualizations also provided multiple perspectives, increasing interpretability.
Further, researchers were able to apply the model through a retrospective simulation at both hospitals.
But there were several limitations to this study, including that T2-weighted or diffusion-weighted scans may not detect specific issues and that the degree of urgency of items in the abnormality category is not defined.
Previously, studies have shown that artificial intelligence can improve the performance of MRIs.
For example, a study published last December showed that MRIs can detect dying tumor cells with the help of AI from a very early stage. This process occurs using quantitative molecular MRI images to measure various tissue properties, along with AI quickly detecting the death of tumor cells and the response to treatment.
Like MRIs, using a computer vision approach to promote drug discovery is potentially applicable. A study explained that defining the binding free energy of a drug candidate shows various implications of how a drug might work. Doing so using machine learning eases the process and provides more rapid results.
Computer vision models have also identified how diseases change the human body. A study explained that using computer vision to analyze images can detect changes in reaction, as precise as changes in individual cells.