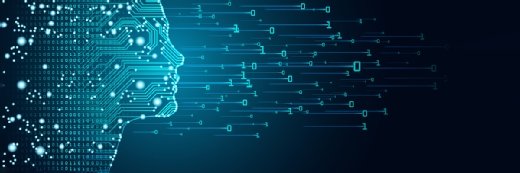
Getty Images
Machine-Learning Model Helped Streamline 22% of Pediatric ED Visits
Machine learning and novel workflows enhanced test ordering processes within a pediatric emergency department, thereby streamlining care, a new study shows.
While exploring the possibilities of integrating machine learning into clinical decision-making, a JAMA Network study found that novel machine learning-driven workflows helped improve test ordering within pediatric emergency departments (EDs).
The study included 42,238 boys up to the age of 18, all of whom came from the emergency department of the Hospital for Sick Children in Toronto, Canada. There were 77,219 patient visits, all of which took place between July 1, 2018, and June 30, 2019.
Machine-learning models predicted the need for services such as urinary dipstick testing, electrocardiogram, abdominal ultrasonography, bilirubin level testing, and forearm radiographs.
Using a variety of statistical analyses, researchers evaluated the models to determine the time between completing patient triage and test use.
Researchers found that using machine learning-based medical directives (MLMDs) can streamline care for 22.3 percent of all patient visits by autonomously ordering common ED tests. These directives also enable test results to be available 165 minutes earlier than usual.
This data shows that the implementation of MLMDs into clinical automation can help care teams obtain test results at a rapid pace. Also, as the use of MLMDs within the ED increases, care teams will be able to expand outreach and care for a more significant number of patients.
Despite these results, researchers acknowledged various limitations that may have affected the research. Primarily, retrieving information from one ED over just one year indicates the use of limited data. Also, researchers admitted that the lack of a cost-effective analysis is an essential factor to consider, as it generally is supposed to occur before use in clinical trials.
The use of machine learning to assist workflow within EDs is not uncommon, with the technology frequently being used to improve clinical decision support.
For example, a study from January explains how the University of Minnesota Medical School evaluated a machine-learning tool following its implementation at a local hospital. Research showed that this tool enhanced clinical decision support, and it was also able to help predict cases of COVID-19.
Artificial intelligence (AI) devices have also assisted in pediatric care. An AI-powered screening system made to assist clinical workflow at the Cincinnati Children's Hospital Medical Center allowed researchers to find patients for various pediatric clinical trials. The system screens EHR data, locating patients for clinical trials faster.
Further, an AI-driven EHR system developed by MIT researchers helped physicians perform various tasks, such as locating medical records, allowing them to spend more time with their patients.