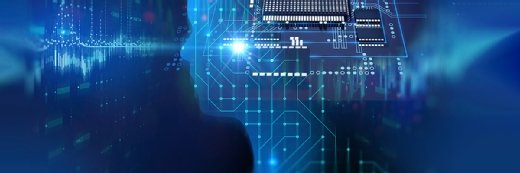
Getty Images
AI Method Helps Further Outcomes-Based Research for Cardiovascular Disease
Utah-based researchers used a new artificial intelligence method, which leverages EHR data, to analyze comorbid diagnoses and demographics that influence cardiovascular disease.
Researchers used University of Utah and Primary Children’s Hospital EHRs to create a Poisson Binomial-based Comorbidity (PBC) method that can help shine a light on the impacts of comorbid conditions and demographics upon cardiovascular health, enabling outcomes-driven research.
As patients develop comorbidities related to cardiovascular disease, these do not remain isolated, but rather combine to create " a complex web of influence on any given outcome," researchers stated.
They used EHR data for over 1.6 million patients and 77 million visits from the University of Utah and Primary Children’s Hospital, studying cases relating to a heart transplant, sinoatrial node dysfunction, and congenital heart disease. The research also included over 500,000 mother-child pairs, used to research cross-generational relationships.
The implementation of the PBC method helped present multimorbidity networks, showing that the method can provide credible data regarding patient demographics, diagnoses, procedures, medications, and how they relate to the disease.
The researchers also implemented probabilistic graphical models (PGMs), which helped them define cross-generational cardiovascular multimorbidities.
"The resulting multimorbidity networks make possible wide-ranging explorations of the comorbid and demographic landscapes surrounding these cardiovascular outcomes, and can be distributed as web-based tools for further community-based outcomes research," researchers stated.
Researchers also noted the limitations of this study. The tactic they used allowed them to examine 30 health conditions at one time, which falls short of their future goals. The inclusion of continuous variables is also a goal for the future, mainly because many software packages today do not allow the inclusion of critical variables.
Contrarily, a considerable strength in this research relates to the ability of this machinery to work as a web-based tool. This will increase the number of users and potentially lead to a new approach to EHR data analysis.
Research shows that the development of AI-based approaches can help identify cardiovascular diseases.
For example, a September 2020 study described a machine-learning model that can locate gut bacteria and use it to pinpoint patients that may have or be at risk of developing cardiovascular disease.
Another cardiologist-developed machine learning model was able to use data regarding coronary artery calcium (CAC) to identify patients that could be at risk for a future heart attack. Researchers conducted this study by selecting patients to undergo CAC scoring and used this information to make predictions through machine learning. During the following 15 years, 76 of the 1,912 patients involved in the study suffered from cardiac death, similar to what the machine-learning model predicted.