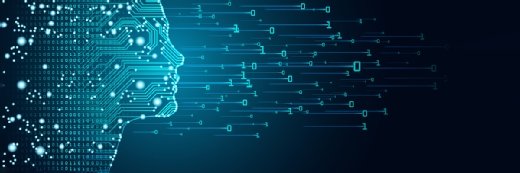
Getty Images
AI to Detect Hip Fracture Outperforms Clinicians, But Use May Be Limited
A new study indicates that a deep learning model can outperform clinicians at detecting hip fractures, but the algorithm’s shortcomings limit its usability in clinical settings.
A deep-learning algorithm outperformed clinicians at detecting proximal femoral fractures, a type of hip fracture, when presented with X-ray images, according to a study published in The Lancet Digital Health.
This is a promising step toward reducing hospitalization and deaths related to the condition. The study assessing this artificial intelligence (AI) algorithm noted that up to 10 percent of patients suspected of having these fractures were not diagnosed following the initial X-ray and required additional medical imaging. Of those who underwent additional imaging, only a third were diagnosed with a fracture.
Delayed diagnoses and additional imaging drive up costs, overburden clinicians and resources, and worsen patient outcomes. Thus, AI models for accurate fracture detection have significant potential for clinical use if developed and deployed effectively.
The deep-learning model was trained to detect hip fractures using a development dataset of over 45,000 proximal femoral X-ray images, with just over 11 percent presenting a fracture. After the model was developed, it was given 400 X-ray images, half of which presented a fracture, from a separate dataset. Thirteen clinicians were given the same images.
The algorithm and clinicians were tasked with classifying the images into various categories based on whether they thought a fracture was present or not present or if more imaging was necessary to determine fracture status.
Overall, the model outperformed the clinicians in terms of detecting fracture presence.
The study also used an algorithmic audit, a method that evaluated the worst mistakes the model could make and any unexpected behaviors it engaged in, rather than the best performance it could achieve. By highlighting the types of cases where the algorithm failed, further research can be directed toward addressing those specific failures.
But limitations within the study and the deep-learning model present significant challenges for its implementation in clinical settings.
For instance, the deep-learning model used was unable to analyze images in which the patient had implanted metalwork from a surgery, the sample size was limited to give the clinician readers a reasonable number of cases to review, and there were no racial identity or ethnicity data attached to the cases used in the study, making it impossible to evaluate the model’s performance when considering groups of varying racial and ethnic backgrounds.
In addition to these limitations, the study indicated multiple shortcomings in the model’s behavior, which may need to be considered when implementing deep-learning algorithms in clinical settings. The model was prone to specific errors, such as misdiagnosing a highly displaced fracture, a type of fracture that would be relatively clear and easily interpreted by a human clinician.
The study indicates that these mistakes may result from how the images are presented to the model or what is or is not highlighted within the image, as in the case of saliency maps. The model also had a higher error rate on cases with abnormal bones caused by disease.
These issues are part of a broader conversation researchers are having surrounding how limitations throughout a study can stymie healthcare-focused machine learning (ML), specifically in medical imaging. However, other research suggests that ML in medical imaging shows promise, including a study showing ML was successfully used to identify hip fractures in the UK.