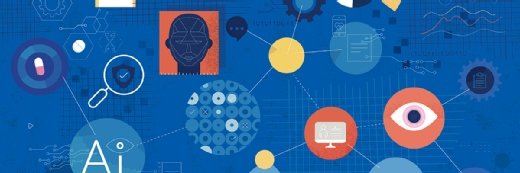
DrAfter123/DigitalVision Vectors
AI Method to Predict Sepsis Mortality Outperforms Conventional Approach
A new study shows that machine-learning algorithms outperform conventional methods for predicting sepsis mortality rates using administrative data.
Machine learning (ML) algorithms that leveraged an administrative database outperformed conventional methods of predicting sepsis mortality rates, according to a study published in the Journal of Medical Internet Research.
The Centers for Disease Control and Prevention (CDC) report that 1.7 million adults in the US develop sepsis in a typical year, and 270,000 of them die as a result. Some patients who survive sepsis also develop permanent organ damage and have a higher risk of other medical conditions, even several years after recovering. Costs incurred from sepsis treatment vary based on the severity of the infection and whether the infection was present at the time of admission to the hospital, but research indicates potential costs between $16,324 and $51,022 per patient. Patients who develop other medical conditions resulting from sepsis incur additional costs.
ML models have previously been applied to point-of-care sepsis mortality prediction, but the new study aimed to compare the performance of ML algorithms to that of conventional prediction models for in-hospital sepsis mortality utilizing administrative data.
The researchers developed four ML models to analyze the data and compared the results with an already existing AI model, the Super Learner model, and the conventional prediction model, the Severe Sepsis Mortality Prediction Model. Data related to demographics, comorbidities, hospital characteristics, diagnosis, and procedure performed on the first day of admission were used as variables for prediction.
The ML algorithms were trained using a dataset of 726,918 adult patients pulled from the US Nationwide Inpatient Sample (NIS) database from 2010 to 2013. They were validated on a dataset of 196,841 adult patients from NIS 2014.
Overall, the ML models outperformed the conventional prediction model. Of the AI algorithms, the gradient-boosted decision tree method and the deep learning neural network model outperformed the others in predicting sepsis mortality.
According to the study, models for predicting sepsis mortality are useful for calculating sepsis risk-standardized mortality rates (RSMRs), which are significant for measuring sepsis care quality across health systems. Gaps between a facility’s RSMR and those of the highest-performing hospitals can help draw attention to differences in practice that could lead to improvements in the quality of sepsis care and allocation of resources.
The researchers also noted that extracting data from hospital administrative databases for research is less time-consuming and more cost-effective than attempting to use data from EMR systems, making prediction models based on administrative data an effective strategy for future predictive algorithms and, thus, measurements of sepsis care quality.
Despite these potential benefits, the researchers state that theirs is the first study that they are aware of to use advanced ML models to predict in-hospital sepsis mortality based on administrative data. They warn that more research is needed before models can be used in hospitals.