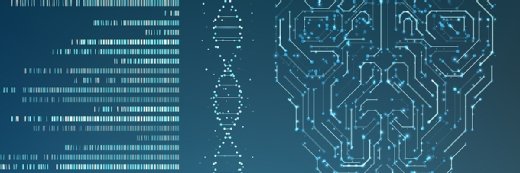
Nobi_Prizue/istock via Getty Ima
Universal Approach Needed to Improve Length of Stay Prediction Models
A unified length of stay predictive analytics framework could address operational issues and improve patient outcomes, but there are several hurdles to developing one, a new study suggests.
A systematic review published in PLOS Digital Health proposed the creation of a unified hospital length of stay (LoS) prediction framework following the analysis of recent developments in LoS prediction approaches and an examination of their successes and shortcomings.
According to the study, LoS is a measure used by hospitals to improve patient care, reduce costs, and allocate resources. LoS has also been used as a measure of patient flow and hospital care unit efficiency. More accurate LoS predictions can improve these outcomes, encouraging researchers to develop better prediction models.
The researchers gathered 93 studies from 1970 to 2019 relevant to LoS prediction. Studies included fell into one or more of the following categories: ones that deal with general adult LoS and specialized LoS, ones that examine one or more features that are related to patient LoS, those that do not deal strictly with research in the medical field related to clinical treatment, studies that do not deal with experiments using animals, and surveys and systematic reviews of LoS prediction methods.
The studies analyzed in the review were then evaluated based on approach type and data used.
Overall, the researchers determined that the approaches and data were often too specific, which has prevented a generalizable framework for LoS prediction from being developed sooner. They further noted that almost all the studies reviewed relied on disease-, condition-or patient-specific approaches, even though LoS is affected by a combination of factors such as patient characteristics, presenting complaints, complications and discharge planning, and treatment complexity. Machine-learning approaches that were evaluated were difficult for clinicians to interpret, which presents problems for clinical implementation and use.
Additionally, many LoS prediction approaches are based on a single hospital or institution and therefore cannot be applied outside that environment. It is also difficult to compare approaches for this reason.
Many of the studies analyzed also consider a physician’s diagnosis of a patient’s presenting complaint, but not the influence of inpatient admission by nurses or other medical staff on predictions of LoS. Nursing admission data and the amount of time nurses spend with patients compared to doctors could highlight other factors relevant to LoS prediction that aren’t fully represented in the existing literature.
The trend of centering LoS prediction model research on urban hospitals presents a similar issue as it does not consider factors unique to patients utilizing rural healthcare systems.
The researchers posit that these issues, along with the growing importance of LoS prediction, indicate the need for a universal prediction model trained using data routinely collected in most health systems. The development of such a model would require that data collection be more consistent, datasets be larger and more diverse, and the resulting approaches be both generalizable across patient populations and interpretable by clinicians, the researchers said.
This recommendation aligns with what experts say is needed for a successful predictive analytics strategy in healthcare.