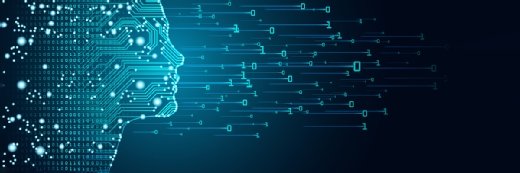
Getty Images
New AI Model Classifies Seizures More Accurately Than Standard Methods
A new deep-learning model developed to detect and classify epileptic seizure subjects has higher accuracy and lower computational time than conventional models, new research shows.
Researchers have developed a convolutional neural network (CNN) model, a type of deep learning model, for classifying epileptic seizures that is designed to provide maximum accuracy and minor computational complexity, according to a study published in Soft Computing.
The researchers developed their algorithm by integrating CNN architecture with a hierarchical attention mechanism, which was expected to enhance the model’s performance. The model comprises three parts: a feature extraction layer, a hierarchical attention layer, and a classification layer.
The model, which also uses a support vector machine (SVM) algorithm, analyzes a feature map obtained from the raw EEG signal and determines whether the EEGs it was taken from are “healthy” or “seizure.”
To test their hierarchical attention-based CNN’s performance, the researchers trained and tested it on a dataset of EEG signals taken from Bonn University. The EEG signals in the database are grouped from A to E, with A and B considered as “healthy,” C and D as “inter-ictal” or the period between two seizures, and E as “ictal” or the period of a seizure.
The results of the hierarchical attention-based CNN’s performance were then compared to the performances of a conventional CNN and an attention-based CNN in three separate trials. On average, the hierarchical attention-based CNN significantly outperformed the other models, achieving a classification accuracy of 97.03 percent, compared to 96.20 percent for the attention-based CNN and 95.65 percent for the conventional CNN.
The models were also evaluated based on their computational complexity, which was measured using the computational time of the algorithms. The hierarchical attention-based CNN outperformed the other models in this area, but all models achieved a maximum run time of less than half of a second.
Other AI models are also being used for EEG and seizure research.
A study from 2020 found that a network inference technique could be used to analyze EEG electrodes to detect a seizure and pinpoint its location in real time. Most seizures are understood to occur when a strong, hyper-synchronized firing of a cluster of neurons suddenly interrupts normal brain activity. If a patient is hooked up to an EEG during a seizure, this abnormal brain activity can be measured and presented as spike-and-wave discharges. However, temporal EEG signals can make it more difficult to detect a seizure.
The researchers developed a network inference technique to study the relationships between EEG nodes to address this.
Their method allows them to detect seizures and pinpoint their locations with significantly more accuracy and fewer resources than conventional approaches, according to the study.