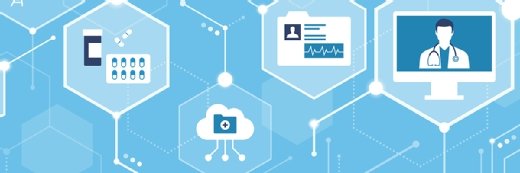
elenabs/istock via getty images
Deep Learning Model Able to Detect Emotion Related to COVID-19
Researchers have developed an emotion detection AI model that could be used to help emergency responders direct people to mental health services during a pandemic, a new study finds.
A new study published in Applied Soft Computing found that the content of emergency response phone calls could be analyzed and classified by a deep learning model based on emotion detection, and then used to assist callers experiencing mental breakdowns.
Emotion detection is a subset of sentiment analysis that aims to extract emotions from text, speech, or images. The researchers posit that emotions determine human behavior, making the development of a method to recognize emotions automatically crucial for use during global crises, such as the COVID-19 pandemic.
The researchers state that a deep learning-driven emotion detection model could be used to help understand people’s emotions, which, in addition to coordination with NGOs and volunteers, would assist those in need during a crisis and help avoid some pandemic-related emotional turmoil.
The research team began by creating a deep learning model designed to detect and classify emotion. Their model, the Average Voting Ensemble Deep Learning (AVEDL) model, was developed by combining the pre-trained transformer-based models BERT, DistilBERT, and RoBERTa.
AVEDL was trained and tested for emotion detection using two datasets.
The first dataset was based on COVID-19 related calls received by the Emergency Response Support System (ERSS) in Kerala, India. Call recordings containing the words “corona,” “COVID-19,” “quarantine,” “COVID,” and “lockdown” from January to March 2021 were randomly selected and transcribed. Each call was annotated and labeled based on emotion by three individuals to avoid bias. Over 20,000 calls were included in the dataset.
The second dataset was made up of 124,740 tweets from an already existing database of COVID-19 related tweets labeled for emotion analysis. AVEDL was trained and tested to detect emotions like sadness, anger, anticipation, fear, or no specific emotion because these made up the majority of the labels in the datasets.
The combined datasets were then divided into training, testing, and validation sets.
When evaluating how successful the models were in distinguishing specific emotions from a group of emotions, the AVEDL model significantly outperformed the other algorithms. The AVEDL model achieved an accuracy of 86.46 percent, while DistilBERT, BERT, and RoBERTa achieved maximum accuracies of 84.86 percent, 84.91 percent, and 85.69 percent. Despite this success, the AVEDL model is limited in that its time complexity is high compared to the others, meaning that its analysis takes longer to complete. The model also performs poorly when using the normal ERSS dataset, which is not related to pandemics.
To combat these limitations, more research is needed, but the researchers posit that the current model has potential as a digital health intervention. They indicate that the model could be used in ERSS call centers to analyze call content in real time to classify emotions, allowing the call center representative to identify callers feeling fear or anger and forward the call to a mental health professional equipped to deal with emotional distress.
The researchers also note that their model has the potential to be used in social media post analyses, allowing automated messaging and education to be provided based on the emotions and attitudes detected in the user’s post. The authors suggest that personalized education interventions could provide emotional support and ensure emotional wellbeing in the event of a future pandemic.