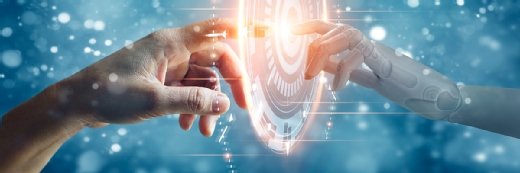
Getty Images
Machine-Learning Models Outperform Clinicians in Predicting Cancer Growth
A new study shows that machine-learning models can utilize natural language processing to predict lymph node metastasis in lung cancer patients.
Researchers have developed lymph node metastasis (LNM) prediction models based on natural language processing (NLP) and machine-learning (ML) algorithms.
In a study published in JMIR Medical Informatics, researchers noted that though LNM is key for treatment decision-making for patients with resectable non-small cell lung cancer, it is difficult to diagnose preoperatively. However, electronic medical records (EMRs) contain a large amount of information related to LNM, including some key information recorded in free text or other unstructured clinical data.
Free text data can be more difficult to normalize, potentially limiting its use in predictive analytics. To combat this, the researchers decided to use NLP to help extract relevant predictive features from computed tomography (CT) reports. Following feature extraction, the data was combined with other structured clinical information from the EMRs and analyzed by six ML models to generate predictions.
EMR data from 794 patients who underwent surgical resection for non-small cell lung cancer at Peking University Cancer Hospital from 2010 to 2018 were included in the study. Specifically, data related to demographic information, medical history, CT reports, preoperative serum tumor markers, and pathology reports were analyzed to develop the prediction models. Clinical staging data that surgeons evaluated before the operation was also collected for each patient to be used as the baseline to compare to the prediction models.
All six ML models achieved high performance in terms of predicting LNM status. Additionally, every model outperformed clinicians’ evaluations based on clinical staging data. It was also determined that the NLP approach could effectively extract features necessary for developing an LNM prediction model.
Despite these successes, the study has significant limitations that need to be addressed before the LNM models see clinical use. External validation of the models is required to further show their effectiveness and generalizability. However, validating the model may be difficult because it was developed using CT reports from only one medical center. Utilizing information from other health systems may pose a challenge for the model due to variations in the writing styles found in free text data.
As artificial intelligence use in healthcare grows, studies have shown that NLP can be used in multiple scenarios to detect disease and enhance care.
In a November 2020 study, researchers used NLP tools to analyze data from pathology reports and identify patients with HPV-related cancers. Most clinical data to diagnose HPV cancers are stored in free text data found within pathology reports, so researchers created an algorithm that could extract this information. When tasked with reviewing 949 pathology reports and identifying abnormal cytology, histology, and positive HPV tests, the NLP algorithm performed on par with clinicians’ evaluations.
NLP can also be used to improve mental healthcare. In an April 2022 scoping review, researchers explored how NLP algorithms have been used to study bipolar disorder.
In numerous studies, the clinical and practical implications of using social media data in bipolar disorder interventions have been evaluated. Some studies suggested that this data could aid early detection, clinical evaluation, and suicide prevention while also complementing existing strategies to address adverse outcomes. Other research indicated that social media and EHR data could be used to flag worsening mental health, create markers for interventions, and help distribute treatment via telehealth.