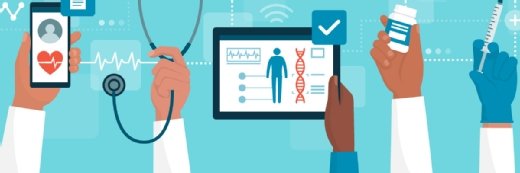
elenabs/istock via getty images
PA Health System Develops ML Model to Interpret Cancer Mutations
Pennsylvania-based Children’s Hospital of Philadelphia has developed a machine-learning tool to help clinicians identify and interpret cancer mutations.
Researchers at Children’s Hospital of Philadelphia (CHOP) have developed a machine-learning (ML) platform to help clinicians identify cancer mutations and interpret their potential significance more efficiently than traditional methods.
The tool, known as CancerVar, is designed to facilitate clinical interpretation of somatic variants, which are DNA alterations in the body’s non-germ cells that can cause cancer and other diseases. The tool can also help reduce clinician labor, improve variant classification consistency, and promote the implementation of the 2017 joint guidelines for somatic mutations proposed by the Association for Molecular Pathology (AMP), the American Society of Clinical Oncology (ASCO), and the College of American Pathologists (CAP).
"CancerVar will not replace human interpretation in a clinical setting, but it will significantly reduce the manual work of human reviewers in classifying variants identified through sequencing and drafting clinical reports in the practice of precision oncology," said Kai Wang, PhD, professor of pathology and laboratory medicine at CHOP in the press release. "CancerVar documents and harmonizes various types of clinical evidence, including drug information, publications, and pathways for somatic mutations in detail. By providing standardized, reproducible, and precise output for interpreting somatic variants, CancerVar can help researchers and clinicians prioritize mutations of concern."
In a study evaluating CancerVar, researchers noted that somatic cancer variant identification, classification, and interpretation could pose significant challenges for cancer diagnosis and prognosis. To date, millions of somatic variants have been identified, and multiple databases have been created to catalog them. However, these databases do not provide standardized interpretations of somatic variants.
The AMP/ASCO/CAP 2017 classification scheme was developed to standardize guidelines for interpreting, reporting, and scoring somatic variants, but the proposal does not specify how to implement these standards. As a result, different databases were providing different results.
To combat this issue, CancerVar combines clinical evidence for 13 million somatic variants from 1,911 cancer census genes mined from existing studies and databases with a deep-learning algorithm that allows clinicians to generate automated descriptive interpretations for variants.
"Somatic variant classification and interpretation are the most time-consuming steps of tumor genomic profiling," said Marilyn M. Li, MD, professor of pathology and laboratory medicine and director of cancer genomic diagnostics at CHOP, in the press release. "CancerVar provides a powerful tool that automates these two critical steps. Clinical implementation of this tool will significantly improve test turnaround time and performance consistency, making the tests more impactful and affordable to all pediatric cancer patients."
The researchers posit that CancerVar shows how computational tools can be used to automate human-generated standardizations and guidelines while also highlighting how ML can bolster clinical decision-making. They also note that other precision medicine software which uses the AMP/ASCO/CAP rules to standardize variant interpretation is available, but these commercial options have high license fees that make them cost-prohibitive for many cancer researchers.
Other ML models to improve cancer care have also been developed recently.
One study has shown that machine-learning models can outperform clinicians in predicting cancer growth. Research indicates that lymph node metastasis (LNM) is key for clinical decision-making for patients with resectable non-small cell lung cancer, but they can be difficult to diagnose preoperatively. To address this, researchers developed lymph node metastasis (LNM) prediction models that leverage natural language processing (NLP) and machine-learning (ML) algorithms.
The NLP algorithm was effective at extracting relevant free-text data from patient EMRs and computed tomography (CT) reports. The data was then given to six ML models, all of which achieved high performance in predicting LNM status. Further, all models outperformed clinicians’ evaluations based on clinical staging data.