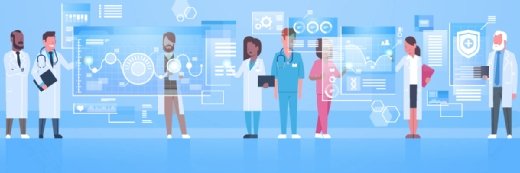
mast3r - stock.adobe.com
New Framework to Evaluate Bias in COVID-19 Prediction Models
Researchers have developed a framework designed to evaluate bias within medical artificial intelligence models and help users address it.
Researchers have developed a framework to evaluate and address unrecognized modeling bias that can be present in healthcare-related artificial intelligence (AI).
According to a study published in JAMIA, bias in AI used within clinical practice can lead to direct harm for patients. Despite this, biases remain incompletely measured in many AI models. For these reasons, the researchers sought to develop a framework to help evaluate and address modeling bias present in medical AI.
The researchers began by studying unrecognized bias in four validated prediction models of COVID-19 outcomes. They performed a retrospective evaluation, to investigate whether the models were biased when developed, and a prospective evaluation, to determine whether the bias changed over time when applying the models on COVID-19 patients who were infected after the models were trained.
Data from 56,590 patients with a positive reverse transcription polymerase chain reaction (RT-PCR) test for SARS-CoV-2 between March 2020 and September 2021 were analyzed. Researchers used 15,000 tests to train and test the retrospective model, while the other 41,000 were used for the prospective model.
The researchers evaluated bias based on race, ethnicity, and biological sex. Bias was also measured across time by comparing the multiple bias metrics against models that were trained on all patients. To get an overall picture of model performance, the researchers used the Machine Learning pipeline for modeling Health Outcomes (MLHO), which can provide comprehensive performance evaluations from different perspectives, including both model-level and individual-level.
When evaluating from the model-level perspective, the researchers did not find consistent bias against all underrepresented groups in the dataset. The individual-level evaluation, however, revealed consistent bias in the form of higher error rates for older individuals. Compared to the models that were trained all patients, the retrospective and prospective models performed slightly worse across time for male patients and better for Latinx and female patients.
The researchers noted that these biases are driven by several factors, including healthcare disparities, data quality issues, social determinants of health (SDOH), and systematic inequalities. These factors can cause certain predictors to be unavailable or make data difficult for an AI to accurately analyze because of data noise. If an AI model has been trained on a certain predictor, and that predictor is unavailable or obscured, the AI won’t be able to predict accurately.
The multifaceted nature of where bias comes from necessitates multiple perspectives to effectively overcome it, researchers concluded. By using their framework, a researcher would be able to evaluate where bias exists and potentially trace it back to its root, where it could then be eliminated.
Developing these frameworks is one of the key aspects of addressing medical data biases, but bias must be addressed in other areas as well.
A study published in npj Digital Medicine earlier this year found that challenges and biases exist at all steps of the research process, which could limit the use of machine learning and other kinds of AI. The researchers reviewed literature and other data related to the use of machine learning in medical imaging. They found that data limitations, evaluation issues, and publishing incentives can slow clinical progress.
Issues related to data can arise due to how they are collected, how datasets are created, and what biases may exist within the datasets. Evaluation presents several challenges, including the appropriate selection of evaluation targets and adoption of statistical best practices. Incentives at the publishing stage, such as using complicated language to impress peers and the pressure to publish manuscripts with “novel” methods and positive results, reduce reproducibility of studies.
To address these problems, the authors suggest raising awareness of data limitations, encouraging established best practices for evaluations, and improving publication expectations around transparency and reporting.