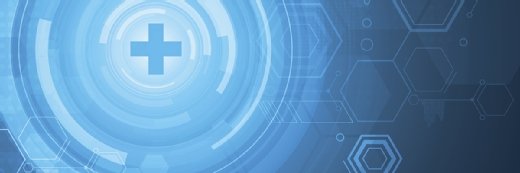
traffic_analyzer/DigitalVision V
Machine-Learning Platform Can Accurately Predict Surgical Complications
Researchers from the University of Florida have successfully developed a machine-learning model to help clinicians predict and address postoperative complications.
University of Florida (UF) researchers have developed a machine-learning (ML) platform, called MySurgeryRisk, that can predict surgical complications as accurately as clinicians.
Surgery and potential postoperative complications provide considerable challenges for clinicians and patients. According to a study published in BMC Surgery last year, the total volume of major surgeries performed annually worldwide was estimated to be 312.9 million in 2012, and an estimated 7-15 percent of these patients would experience a major complication.
The researchers created MySurgeryRisk to help prevent some of these outcomes by predicting risk rates for patients planning to undergo surgery. The platform was developed using 74,000 procedures involving 58,000 adult patients at UF Health, and it was trained to predict surgical complications using 135 variables extracted from patient EMRs. These variables included clinical, laboratory, pharmacy, demographic, and other data.
The algorithm was then tasked with predicting prolonged intensive care unit stays and mortality risk after procedures. The platform also predicts the risk of eight major surgical complications, including neurological issues, cardiovascular issues, sepsis, and acute kidney injury.
Following development and training, the researchers compared MySurgeryRisk’s ability to predict complications in 100 cases to surgeons' predictions for the same cases. The algorithm performed on par with the surgeons in predicting the probability of neurological complications, delirium, sepsis, and prolonged mechanical ventilation, but it outperformed them at predicting blood clots in veins.
After processing the data and making predictions, the tool transmits results to surgeons’ mobile devices to support clinical decision-making. The researchers note that predicting a higher complication risk for a patient planning an operation could lead to patient-clinician conversations about whether surgery is appropriate. These predictions are also beneficial for clinicians with less experience in predicting complications.
Further, the tool is valuable for surgeons as they prepare to begin a surgical procedure. With risk predictions from MySurgeryRisk in mind, surgeons can be more strategic in the operating room, potentially choosing to implement additional techniques, such as individualized blood pressure management, to improve patient outcomes.
MySurgeryRisk was successfully validated in two prior studies. In 2019, researchers compared the tool’s predictions to those of 20 physicians, which showed that the platform achieved high performance. The second study tested MySurgeryRisk’s accuracy in predicting the previously mentioned eight postoperative complications among over 51,000 surgical patients at UF Health Shands Hospital between 2000 and 2010. The tool predicted complications with an accuracy ranging from 70 percent for wound complications to 80 percent for sepsis.
In the future, the researchers aim to make MySurgeryRisk more precise by including in its predictions some of the thousands of potentially relevant, uncollected data points found in the operating room, such as information from anesthesia devices and ventilator monitors. They also hope to expand validation testing of the tool at other health systems across Florida and make it more widely available.