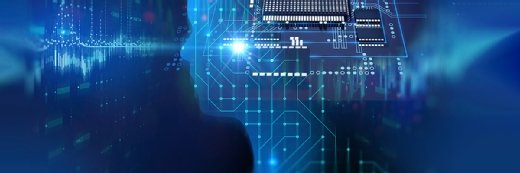
Getty Images
AI Models Accurately Predict Clinical Risks in Multiple Hospitals Using Live Data
Researchers have shown that clinical risk prediction models for multiple conditions implemented in different hospitals achieve high performance when integrated into live clinical workflows.
A new study published in the Journal of Medical Internet Research earlier this month showed that clinical risk prediction models for sepsis, delirium, and acute kidney injury (AKI) achieve high performance when implemented into live clinical workflows at multiple hospitals.
According to the study, machine learning (ML) models are used for many types of clinical risk prediction, but most are developed using retrospective data. Few models use data from live clinical workflows or report model performance for different hospitals. The researchers in this study aimed to evaluate the performance of prediction models for sepsis, delirium, and AKI using retrospective data compared to those using live clinical workflow data from three different hospitals.
The researchers noted that prediction models using data from live clinical workflows are crucial due to lack of interoperability in legacy systems used to store retrospective data and differences in the prevalence of various clinical risk events. These can negatively affect the accuracy of a model’s predictions, which can then impact quality of care and outcomes for these risk events.
The researchers began by training prediction models for the three use cases using retrospective data from each hospital. They trained each retrospective model using a calibration tool common for all hospitals and use cases. Then, they built and trained new models for use in live clinical workflows and calibrated those models with each hospital’s specific data. These new models were then deployed in the hospitals and used in regular clinical practice. Their performance was compared with the models using only retrospective data for each hospital, and the researchers also conducted a cross-hospital evaluation by generating predictions for one hospital using a model trained on another hospital’s data.
Overall, the performance of the prediction models using data from live clinical workflows was similar to that of the models using retrospective data. However, the cross-hospital evaluation showed severely reduced prediction performance. This decline in performance highlighted limitations in developing generic prediction models for use in different hospitals, the researchers noted.
These findings indicate that model calibration using data from the deployment hospital is crucial for a prediction model’s success. The models utilized in the study were all developed using a generic process, but what guaranteed their high performance was the calibration step that utilized specific hospital data, the researchers noted.
When the prediction models were being used in the hospitals, clinicians were also able to provide feedback surrounding model usefulness and performance after they closed a given prediction alert. There were 134 feedback entries collected for AKI use cases at one of the hospitals, and 34.3 percent of these indicated that the user found the predictions useful. A total of 27.6 percent of predictions were considered to be false positives by clinicians, and in 38.1 percent of the cases, the end users were already aware of the AKI risk raised by the alert.
The researchers concluded that using a generic model development process and implementing calibration tools to generate hospital-specific models is a valid approach to prediction model design and guarantees model performance in different hospitals. However, research focused on evaluating the detailed clinical outcomes of prediction models in medical practice is needed.