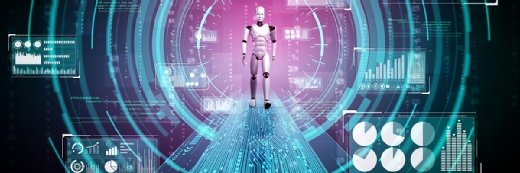
Getty Images
Machine-Learning Models Can Predict Sepsis-Related Mortality
Researchers have developed machine-learning models that can accurately predict 30-day mortality in patients with sepsis-associated encephalopathy.
A new study published in BMC Medical Research Methodology highlights how researchers developed and trained machine-learning (ML) models that can accurately predict 30-day mortality in patients with sepsis-associated encephalopathy (SAE).
SAE is a common neurological complication related to sepsis, according to the National Institutes of Health (NIH). It is poorly understood because of its pathophysiology. The condition is typically characterized by diffuse brain dysfunction secondary to infection elsewhere in the body without overt central nervous system infection, but a diagnosis can usually only be made after other conditions are ruled out. SAE has a high mortality rate, and survivors tend to develop further neurocognitive impairment in the future.
These adverse outcomes underscore the need for researchers to identify key predictors of SAE-related mortality and implement them in the development of predictive models, the study authors noted. To develop these models, the researchers gathered retrospective data from the publicly available Medical Information Mart for Intensive Care (MIMIC)-IV dataset, which contained de-identified data from 383,220 admissions to the intensive care units (ICUs) at the Beth Israel Deaconess Medical Center between 2008 and 2019.
Patients with a diagnosis of sepsis as defined in the Third International Consensus Definitions for Sepsis were pulled as part of participant selection. Of these, patients under the age of 16, with ICU stays of less than 48 hours, with primary brain injury, pre-existing liver or kidney failure affecting consciousness, chronic alcohol or drug abuse, and severe electrolyte imbalances were excluded from the study. A total of 6,994 patients were included in the final cohort, with data from 4,897 being used to train the models and data from 2,097 to validate them.
The researchers also used recursive feature elimination (RFE), a resource selection method, to select the most relevant variables for predicting SAE mortality. Fifty-six variables were selected and considered in groups of 15, 25, 35, 45, and 56 to evaluate predictor strength.
Overall, 15 variables were determined to have the highest prediction strength: acute physiology score III (APSIII), Glasgow coma score (GCS), sepsis-related organ failure assessment (SOFA), Charlson comorbidity index (CCI), red blood cell volume distribution width (RDW), blood urea nitrogen (BUN), age, respiratory rate, PaO2, temperature, lactate, creatinine (CRE), malignant cancer, metastatic solid tumor, and platelet (PLT). These variables were then used in all training and testing sets for every ML model.
All nine models achieved high performance, with the artificial neural network, the logistic regression model, and the adapting boosting model demonstrating the highest prediction accuracies. The researchers also developed a web-based calculator to make it easier for surgeons to use the models.
These findings show that ML models could be used to effectively evaluate the death risk of SAE patients in the ICU, the researchers concluded. Further, they stated that the online calculator could help correct clinical decisions and support timely treatment strategies in clinical settings. However, more research must be done to investigate the long-term prognosis of SAE and its underlying mechanisms.