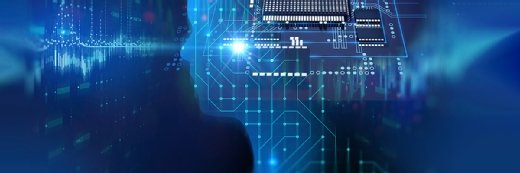
Getty Images
Deep Learning Effective at Predicting Cerebral Palsy in Infants
Researchers have developed and validated a deep-learning model capable of accurately predicting cerebral palsy using spontaneous movements in high-risk infants.
In a prognostic study published in JAMA Network Open, researchers described the development of a deep-learning (DL) model that can effectively predict cerebral palsy based on infants’ spontaneous movements at nine to 18 weeks of age.
According to the Centers for Disease Control and Prevention (CDC), cerebral palsy is a group of disorders that affect a person’s ability to move and maintain balance and posture. Cerebral palsy is the most common motor disability in childhood and is caused by abnormal brain development or damage to the developing brain that affects a person’s ability to control their muscles.
Along with problems related to movement and posture, cerebral palsy patients may also experience seizures, spine changes, joint problems, intellectual disability, and problems with vision, hearing, or speech. Early signs of cerebral palsy in children are usually delays in reaching motor or movement milestones, in addition to more age-specific symptoms.
In the study, the researchers noted that cerebral palsy is typically diagnosed between 12 and 24 months, with more mild presentations of the condition often diagnosed in early childhood. However, the early identification of infants at high risk for cerebral palsy is key for providing targeted interventions and minimizing complications, they stated.
Currently, the most accurate clinical method to predict cerebral palsy in infants younger than five months is the general movement assessment (GMA) tool, which uses characterizations of infants’ general movements, which are spontaneous movements involving the whole body. The GMA tool requires training to be effectively used as it is based on clinical experts’ observations of infants’ general movements in video recordings. Because of this, the experience level of the GMA rater may alter the test’s reliability, hampering widespread clinical use, according to the study.
These limitations spurred the researchers to develop a DL model that could accurately predict cerebral palsy as a potential alternative to the GMA tool. They studied 557 infants with a high risk of perinatal brain injury who were enrolled in previous studies conducted at 13 hospitals in Belgium, India, Norway, and the US between Sept. 10, 2001, and Oct. 25, 2018. Infants included in the study had available general movement video recorded from nine to 18 weeks of age, available classifications of movements ascertained by the GMA tool, and available data on cerebral palsy status at 12 months or older.
A total of 418 infants, or 75 percent, were randomly assigned to the model development sample, which consisted of training and internal validation, while the remaining infants were randomly assigned to the external validation sample. Using these infants’ general movement recordings, the DL model was tasked with predicting cerebral palsy between 12 to 89 months. The predictions were performed automatically from a single video for each infant. The model also predicted additional measures, such as associated functional level and cerebral palsy subtype. The DL model’s performance was compared with a conventional machine-learning model and the GMA tool.
The DL method achieved 90.6 percent accuracy compared to the conventional machine-learning method, which achieved 72.7 percent accuracy, indicating a significant performance improvement. However, there was no significant improvement in accuracy when the DL model was compared with the GMA tool, which achieved 85.9 percent accuracy.
Additionally, the DL prediction model had higher sensitivity among infants with non-ambulatory cerebral palsy compared to ambulatory and among infants with spastic bilateral cerebral palsy compared to spastic unilateral.
These findings indicate that it is possible to develop a DL model for predicting cerebral palsy at nine to 18 weeks of age that achieves significant predictive accuracy on external validation, the researchers stated. This suggests possible avenues for DL–based software to provide objective early detection of cerebral palsy in clinical settings.