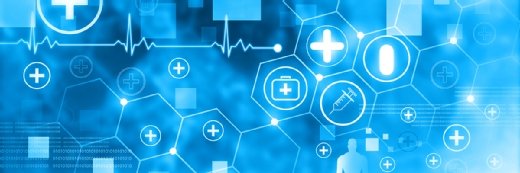
Rasi Bhadramani/istock via Getty
Artificial Intelligence Improves Endoscopy Detection Rates, Reporting
An artificial intelligence tool used to review small bowel capsule endoscopy results was found to increase detection rates of small bowel findings and reduce endoscopy video reading times.
A new study in JAMA Network Open found that the use of an artificial intelligence (AI)-based tool designed to review videos of small bowel capsule endoscopy (SBCE) resulted in a higher detection rate of small bowel findings and reduced video reading times for SBCE.
According to the study, experienced healthcare professionals spend an average of 50 to 120 minutes reading and reporting on full-length capsule endoscopy video recordings. Reading these recordings is a tedious task because it is monotonous but also highly demanding, the authors stated. Thus, clinicians must block out dedicated time slots for review without distractions, which can be difficult.
For these reasons, researchers are becoming increasingly interested in how AI may be able to alleviate the clinician burden in this area. Previous research has found that a convolutional neural network-based algorithm can achieve gastroenterologist-level identification of small bowel diseases and normal variants, but this research is limited, the study authors noted.
To expand the research in this area, the study authors sought to develop and evaluate a CNN-based computer-aided detection algorithm’s ability to detect and classify 17 types of small bowel findings based on capsule endoscopy structured terminology (CEST).
The researchers began by collecting 6,097 de-identified SBCE examinations performed in 51 Chinese medical centers between Jan. 1, 2012, and June 30, 2020. Of these, 272 examinations were excluded due to missing data, leaving data from 5,825 SBCE examinations comprising 295 million images. These data were divided into a training set, which consisted of 2,927 SBCE examinations, or 148 million images, collected from 29 medical centers, and a validation set, which consisted of 2,898 SBCE examinations, or 146 million images, collected from 22 medical centers.
These data were then given to the CNN, known as SmartScan, which was tasked with interpreting the images, and its performance was compared to a conventional reading (CR) of the images. Overall, the SmartScan-assisted reading detected 5,834 small bowel findings, significantly higher than the CR, which detected 4,630 findings. The SmartScan-assisted reading also achieved a higher per-patient detection for CEST findings, with a rate of 79.3 percent, compared with the CR, which achieved a rate of 70.7 percent.
With SmartScan, the average number of images per SBCE video requiring review was reduced to 779.2, compared with 27,910.8 with the CR, for an average reduction rate of 96.1 percent. The average video reading time was also reduced by using SmartScan. The CNN had a reading time of 5.4 minutes compared with the CR, which had a time of 11.6 minutes, resulting in a time reduction rate of 89.3 percent with the SmartScan.
These findings suggest that a well-structured computer-aided detection algorithm, based on CEST, may change the human-based reading and reporting of SBCE videos because the algorithm is associated with an increased detection rate of small bowel findings and reduced SBCE video reading time, the authors stated.