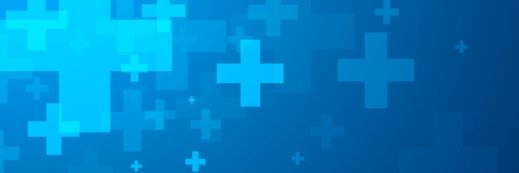
Getty Images
Researchers Propose Framework to Ensure Equitable Healthcare Algorithms
Rand Corp. researchers argue that including race and ethnicity information, rather than removing it, is key to eliminating algorithmic bias.
In new commentary published in Health Affairs, researchers from the Rand Corporation posit that having knowledge of race and ethnicity, and including that data in healthcare algorithms, is necessary to combat algorithmic bias effectively, rather than taking a “race-blind” approach.
The authors further argue that when race and ethnicity are taken into account, various methodological approaches can be used ensure equitable algorithmic performance. When these data are unavailable, imputing them can enhance opportunities to identify, assess, and combat algorithmic bias in clinical and nonclinical settings, they said.
To illustrate their points, the authors describe two applications in which the imputation of race and ethnicity data has the potential to reduce algorithmic bias: equitable disease screening algorithms using machine learning and equitable pay-for-performance incentives.
Currently, there is significant research emphasis on leveraging “big data” from EHRs to develop machine-learning (ML) algorithms that support clinical decision-making. Many of these algorithms are designed to detect or predict medical conditions and outcomes, such as sepsis-related mortality, pancreatic cancer, and postpartum hemorrhage.
Because these tools affect decisions about who receives care, the type of care they receive, and how the care is provided, it is critical that they do not create or perpetuate biases. Racially or ethnically biased algorithms have significant potential to perpetuate worse outcomes for patients who are already at risk for receiving poorer quality care. In the case of a clinical decision support tool for a life-threatening condition, identifying and correcting bias before deployment is crucial.
However, these tools typically rely on data from patient EHRs, which may contain race and ethnicity data for some patients, but not others, or may have incomplete data as a result of a third party reporting the data or of race and ethnicity response options being too limited. In these cases, assigning race and ethnicity data through one of multiple validated methods can ensure that the algorithm is accurately assessed and adjusted for inequitable performance, the researchers state.
Creating equitable pay-for-performance or value-based payment strategies has similar challenges. Pay-for-performance strategies are designed to incentivize and enhance healthcare quality, but can have the opposite effect, exacerbating disparities for certain groups if these strategies are not assessed for bias and corrected, if necessary.
The authors state that healthcare providers who perform poorly on incentive payout measures are more likely to care for disadvantaged patient populations, meaning that these providers receive smaller reimbursements on average. These smaller reimbursements can prevent providers from making investments to improve care quality and delivery, which may prevent them from keeping pace with other providers.
Therefore, approaches to enforce algorithmic fairness can be used to make sure that payments to providers are not correlated with the racial and ethnic composition of patient populations, the researchers argue. However, these approaches require either knowing or imputing race and ethnic data of a given population.
Once this data is made available or imputed, providers can be categorized into multiple groups based on the estimate racial and ethnic composition of their patient population. Then, performance-based payments could be adjusted to ensure that the total payments directed to providers within each group are equal.
Despite the potential utility of imputed race and ethnicity data, the authors indicate that there are limitations to the methodology. For example, even when imputation methods are highly accurate, some uncertainty about the race and ethnicity of a patient will always be present. Because of this, the authors do not recommend using imputation to make individual-level decisions. In individual cases, self-reported data is the gold standard.
Imputation is also limited as a method to ensure algorithmic fairness and equity in the case of multiracial people, who make up a growing proportion of the US population. The researchers note that most imputation approaches are not designed with multiracial people in mind, but future methodologies could address this by allowing people to have noncategorical race and ethnicity designations.
But overall, the inclusion of race and ethnicity, whether reported or imputed, is still better than “race-blind” approaches for ensuring equitable algorithms, the authors conclude.