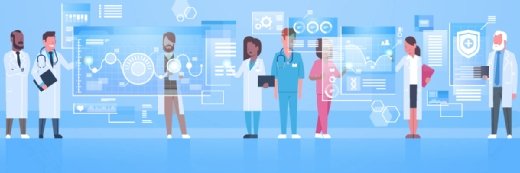
mast3r - stock.adobe.com
Deep Learning May Improve Identification of Brain Tumors
Researchers have found that a deep learning tool may improve brain tumor classification and diagnosis by neuroradiologists.
New research published in JAMA Network Open indicates that a deep learning tool trained to identify and classify brain tumors based on standard MRIs may aid neuroradiologists in diagnosis.
The researchers set out to investigate how deep learning could be used to support brain tumor classification and diagnosis. MRIs and biopsies, the study notes, are commonly used for the diagnosis of these tumors, with biopsy considered the standard for identifying tumor type.
However, since biopsies are invasive, the researchers wanted to develop a potential diagnostic that relies on MRI scans. Unfortunately, identification of tumor type via MRI alone can be difficult for clinicians, so the research team developed a deep learning model to analyze the MRI data.
The model was created using MRI scan data collected between 2000 and 2019 from 37,871 patients. The system was then trained to evaluate each image to classify 18 different types of intracranial tumors. Following training, the model’s diagnostic accuracy was tested on one internal and three external independent datasets. The clinical utility of the model was assessed by comparing its accuracy to that of neuroradiologists.
The model achieved high accuracy, sensitivity, and specificity overall. It also outperformed neuroradiologists in terms of accuracy and sensitivity, but achieved similar specificity. In addition to being compared with the model’s performance, neurologist performance was further evaluated based on their accuracy while assessing tumors while using the model as a support tool versus without its assistance.
Here, the researchers found that with the assistance of the model, neurologists’ average accuracy increased by 12 percentage points, from 63.5 percent without assistance to 75.5 percent with assistance.
The research team concludes that these findings suggest that a deep learning-based automated diagnostic tool may help improve the classification and diagnosis of intracranial tumors among neuroradiologists using MRI data.
This study is one of the more recent efforts to use artificial intelligence (AI)-based approaches to medical imaging and tumor detection.
In 2020, the Perelman School of Medicine at the University of Pennsylvania (Penn Medicine) partnered with Intel to develop a machine learning model to identify brain tumors while safeguarding patient privacy. The model was developed using federated learning, which allows algorithms to be trained using locally-held data across multiple decentralized servers without the need to exchange that data.
In March, computer vision models were developed to improve the quality of head MRIs, which reduce the time taken to report an abnormal head exam and can improve outcomes. These quality enhancements are especially critical within the contexts of brain tumors and traumatic brain injury, in which early detection is a major factor influencing patient outcomes.
These studies show that there is potential for AI to transform medical imaging and clinical decision support, but significant challenges still remain.