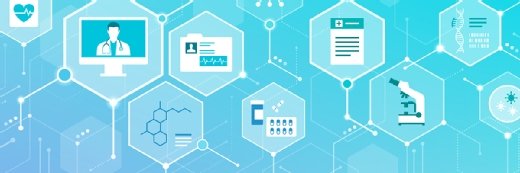
elenabs/istock via getty images
Machine Learning Models Predict Liver Cancer Treatment Response
Researchers have found that imaging-based machine learning models can accurately predict patient response to liver cancer treatment.
A proof-of-concept study published in the American Journal of Roentgenology shows that machine learning (ML) models can predict tumor recurrence in patients with early-stage hepatocellular carcinoma (HCC), a type of liver cancer, prior to liver transplant or related treatments.
Liver cancer creates a significant healthcare burden in the US and worldwide. The CDC reports that each year in the US, approximately 25,000 men and 11,000 women get diagnosed with liver cancer, and about 19,000 men and 9,000 women die from the disease.
HCC is the most common type of liver cancer, according to the Mayo Clinic, and most often occurs in patients with chronic liver diseases. Treatments for HCC are determined by the size and location of a patient’s tumor(s), how well their liver is functioning, and their overall health.
Potential treatments can include resection surgery to remove the cancer, liver transplant, ablation procedures, chemotherapy or radiation therapy, targeted drug therapy, and immunotherapy, in addition to newer treatments for patients participating in clinical trials.
Patients with early-stage HCC are often eligible for resection, transplant, or ablation, but risk of tumor recurrence is a major factor in choosing a treatment and determining potential transplant eligibility, the researchers note. Tumor recurrence is associated with poor health and survival outcomes, making accurate recurrence prediction critical.
Currently, the study states, forecasting recurrence is difficult, and typically relies on biomarker analysis. The researchers sought to evaluate the use of ML models to predict recurrence using pretreatment lab, clinical, and MRI data from early-stage HCC patients who initially met the existing criteria for liver transplant.
The research team collected retrospective data from 120 of these patients diagnosed from June 2005 to March 2018 who underwent treatment via thermal ablation, resection, or liver transplant. Prior to treatment, all patients underwent MRI, and following treatment, patients were subject to imaging surveillance. Clinical and lab data were collected before and after treatment.
To evaluate which data would be best for generating predictions, the researchers created three ML models based on what type of data each used: a clinical model, an imaging model, and a combined data model. Features to be used for prediction were pretreatment MRI and clinical data extracted by a pre-trained feature-selection convolutional neural network.
Each model was assessed based on its ability to predict tumor recurrence within six time frames, ranging from one through six years following treatment. During that time, tumors recurred in 36.7 percent of the study cohort. All models achieved high performance in predicting recurrence, but the imaging model outperformed the model relying only on clinical data. However, the combined model performed on par with the imaging model.
These findings suggest that ML models can predict recurrence prior to treatment in patients with early-stage HCC who are eligible for liver transplant, the researchers state. The application of such models may help enhance criteria for organ allocation and liver transplant eligibility, they conclude.
Other models have also been developed to forecast HCC treatment response.
Last year, Cleveland Clinic researchers developed a deep learning model to predict health and survival outcomes for HCC patients following liver transplant, which achieved significant results.
Artificial intelligence (AI) and ML are also being used to improve timeliness and diagnosis of HCC treatment.
Earlier this month, researcher found that an EMR-based automated care coordination tool could successfully identify and track HCC cases, which had a significant impact on early diagnosis. The tool is designed to review all liver radiology reports, generate a queue of abnormal cases for review, and maintain a queue of cancer care events with due dates and automated reminders.
Using these data, the system sent reminders for follow-up testing and treatment to clinicians, decreasing time from imaging to diagnosis and time from diagnosis to treatment.