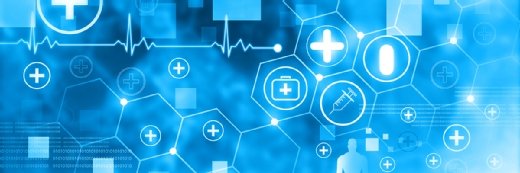
Rasi Bhadramani/istock via Getty
Collaborative Approach to Developing Radiography ML Helps Quantify Joint Damage
A crowdsourcing approach to developing machine-learning models to quantify joint damage via radiographs was proved successful in a new study.
A new study published in JAMA Network Open last week shows that machine-learning (ML) models developed using a crowdsourcing approach can improve efforts to quantify radiographic joint damage in patients with rheumatoid arthritis (RA).
Mayo Clinic reports that RA is a chronic inflammatory disease and autoimmune disorder that affects the linings of the joints, causing painful swelling, stiffness, and, in some cases, physical disability. The inflammation associated with RA can not only cause complications like joint deformity and bone erosion over time but can also impact other parts of the body, such as the skin, eyes, lungs, heart, and blood vessels.
These outcomes make the accurate and timely assessment of joint damage crucial to patient care and treatment decisions. Artificial intelligence and ML have shown significant potential to improve medical imaging, chronic disease management, and clinical decision support in recent years. However, various challenges and limitations, particularly within radiology and radiography, have slowed progress in this area for conditions like RA.
To encourage research, the study authors designed and implemented an international crowdsourcing competition to develop ML models capable of quantifying joint damage using radiographs of RA patients’ hands and feet. Joint damage was quantified in each model using joint space narrowing and erosion.
For the competition, known as the Rheumatoid Arthritis 2–Dialogue for Reverse Engineering Assessment and Methods (RA2-DREAM Challenge), participants used existing radiographic images from two separate clinical studies, which included a total of 674 radiographic sets from 562 patients. The participants were tasked with utilizing this data to develop ML models that could successfully complete three quantification-related sub-challenges: evaluating overall damage, joint space narrowing, and erosions.
The competition received 173 submissions from 26 participants or teams in seven countries for the leaderboard round. Thirteen submissions were included in the final evaluation. These were compared to a baseline model designed to provide a performance benchmark. Models were also compared to expert-curated Sharp-van der Heijde (SvH) scores, a standard method for scoring RA radiographs.
The performance and reproducibility of each model were assessed using a post-challenge independent validation dataset. Overall, the winning models achieved high performance in terms of accuracy and reproducibility. They also produced scores similar to the expert-curated SvH ones.
These findings indicate that the algorithms produced through this crowdsourcing challenge could provide quick and accurate methods to quantify RA-related joint damage, the researchers concluded. Further, they noted that these methods could help improve RA joint damage research and support treatment decisions if integrated into the clinical settings.
This research is among the latest to focus on improving ML models used in medical imaging.
Last week, researchers outlined best practices for mitigating bias in AI and ML tools used in radiology in a three-part special report.