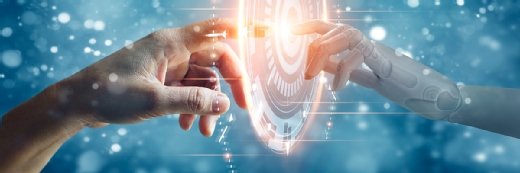
Getty Images
New Machine-Learning Tool Identifies Injection Drug Use Using EHR Data
An automated tool developed by UCLA Health uses patients’ EHR data to detect signs of injection drug use faster than traditional methods.
Researchers at the University of California, Los Angeles (UCLA) Health have developed an artificial intelligence (AI)-based tool that can identify people who inject drugs using EHR data faster and more accurately than standard methods.
According to the study, the efficient identification of people who inject drugs is crucial to improving risk assessment and mitigation, clinical decision-making, and health services research. Currently, identifying this population relies on various International Classification of Diseases (ICD) codes because there is no ICD code specifically for injection drug use.
Because there is no ICD code for injection drug use, providers and coders are forced to rely on other, non-specific proxy codes and data, which can lead to inaccuracies and poorer outcomes, the researchers stated. To address this, they designed machine-learning (ML) and natural language processing (NLP) algorithms to identify people who inject drugs quickly and more accurately than current methods, which rely on manual record reviews.
“By using natural language processing and machine learning, we could identify people who inject drugs in thousands of notes in a matter of minutes compared to several weeks that it would take a manual reviewer to do this,” said study lead author David Goodman-Meza, MD, assistant professor of medicine in the division of infectious diseases at the David Geffen School of Medicine at UCLA, in the press release. “This would allow health systems to identify [people who inject drugs] to better allocate resources like syringe services programs and substance use and mental health treatment for people who use drugs.”
To develop the algorithms, the researchers manually reviewed 1,000 medical records from patients admitted to Veterans Administration (VA) hospitals between 2003 and 2014 with Staphylococcus aureus bacteremia, a common type of infection that occurs when the bacteria enters the body through openings in the skin, such as those found at injection sites.
Following algorithm development and training, the researchers compared the NLP/ML models with 11 models based on proxy combinations of ICD codes. The best-performing models in the study were determined by multiple common statistical metrics, including F-score, sensitivity, and positive predictive value.
Overall, the random forest-based NLP/ML algorithms were the best-performing algorithms in the training set, outperforming all the ICD-based algorithms. The best random forest algorithms achieved an F-score of 0.905, while the best ICD algorithm achieved an F-score of 0.592. The best NLP/ML algorithm had a sensitivity of 92.6 percent and a specificity of 95.4 percent.
These findings indicate that NLP/ML algorithms may be more effective at identifying those who inject drugs than some standard methods, the researchers concluded. Because of this, they argued that such models should be considered for identifying this population to improve clinical decision-making, health services research, and administrative surveillance.
However, the researchers noted that their research has multiple important limitations. As in many studies that rely on EHR data, their study could have been impacted by poor provider documentation.
Further, the dataset for this study spans 2003 to 2014, meaning that it may not reflect recent shifts in injection drug use trends, such as the move from prescription opioids and heroin to synthetic opioids, like fentanyl. Thus, the algorithms in the study may miss cases involving synthetic opioids.
The real-world implications of missing these cases are exacerbated by new findings published last month, which show that only 5 percent of drug overdose patients admitted to the emergency department are tested for fentanyl and synthetic opioids, despite these drugs being the leading cause of death for Americans under 45.