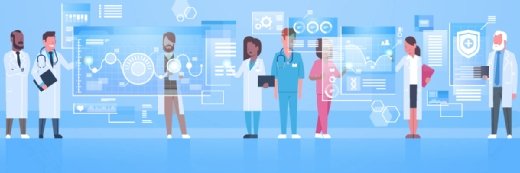
mast3r - stock.adobe.com
Machine Learning Finds New Patterns of Decline in ALS, Alzheimer's
An MIT machine-learning method has found new patterns of disease progression in ALS and other neurodegenerative diseases.
Researchers from the Massachusetts Institute of Technology (MIT) have developed a machine-learning (ML) tool capable of identifying new patterns of health decline in neurodegenerative diseases such as amyotrophic lateral sclerosis (ALS), Alzheimer's, and Parkinson’s disease.
Neurodegenerative diseases are typically complex, associated with various symptoms and progressing at different rates. According to the press release, ALS is a challenge for providers and researchers because its manifestations can vary significantly.
While the disease affects voluntary muscle movement and is always fatal, some patients experience slower disease development, with onset in the limbs and impacts on fine motor skills. Others experience more severe disease development that significantly hampers swallowing, breathing, speaking, and mobility. Likewise, there are also variations in the quality of life and outcomes among ALS patients, the press release notes. Most ALS patients live only a few years after diagnosis, while others survive for decades.
These complexities and variations make understanding the progression of neurodegenerative diseases like ALS, Alzheimer's, and Parkinson’s crucial for enrollment in clinical trials, the discovery of disease causes, and the analysis of possible interventions and treatments. Thus, MIT researchers developed a method to better characterize ALS progression.
“There are groups of individuals that share progression patterns. For example, some seem to have really fast-progressing ALS and others that have slow-progressing ALS that varies over time,” said Divya Ramamoorthy, a research specialist at MIT and lead author of the study, in the press release. “The question we were asking is: can we use machine learning to identify if, and to what extent, those types of consistent patterns across individuals exist?”
The researchers note in the study that assessing the evolution of neurodegenerative diseases can be challenging for many reasons. For example, many studies and clinical trials assume that health declines in a linear, downward trajectory. However, some research indicates that this is not always true for ALS. Data used for this research can also be sparse, subjective, or low-quality.
To build their model and help address these challenges, the researchers relied on five longitudinal datasets from ALS clinical trials and observational studies. They used the gold standard to measure symptom development, known as the ALS functional rating scale revised (ALSFRS-R). They also incorporated survivability probabilities, forced vital capacity, which is a measurement of respiratory function, and subscores of ALSFRS-R, which looks at individual bodily functions.
Following model training, the researchers tested their model against other common approaches. The model identified four dominant patterns of disease: sigmoidal fast progression, stable slow progression, unstable slow progression, and unstable moderate progression. These showed strong nonlinear characteristics, and the model was able to capture trajectories where patients experienced a sudden loss of ability, called a functional cliff, which would significantly impact treatments, enrollment in clinical trials, and quality of life, according to the press release.
Overall, the model outperformed all other approaches and was also found to be applicable to Alzheimer’s and Parkinson’s diseases.