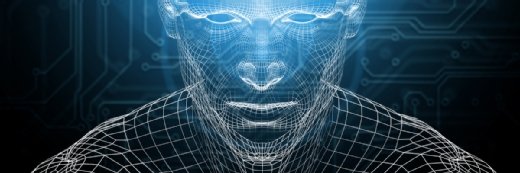
Getty Images
Predictive Analytics Models Determine Diabetes Life Expectancy
Clinicians can use patient data to build predictive analytics models and forecast the five- to ten-year life expectancy of older people with diabetes.
Building predictive models with patient data – such as history of comorbidities and medications – can determine the five- to ten-year life expectancy of older people with diabetes and help providers develop personalized treatment plans, according to a study published in Diabetes Care.
Federal and professional society clinical practice guidelines recommend that diabetes treatments be individualized for older adults, the researchers noted. Critical factors that impact diabetes treatment goals include co-occurring health conditions, such as severe mental illness or cancer, as well as diabetes complications like chronic kidney disease and heart failure. Co-existing conditions and life expectancy also impact treatment goals.
The benefits of lower blood sugar can take years to occur, the team said. For some older adults with limited life expectancy, the treatment burden may not be worth the benefits.
Because of the uncertainty of determining life expectancy for individual patients, researchers sought to build predictive analytics tools that could help forecast mortality in older adults with diabetes.
“The guidelines don't give doctors guidance for how to decide whether or not people fall into these different bins for life expectancy," said Kevin Griffith, research analyst at the Department of Veterans Affairs (VA) Boston Healthcare System.
"Furthermore, clinicians are notoriously inaccurate in predicting life expectancy, with studies frequently showing both over- and underestimating. We developed models with high predictive validity of future mortality in a large sample of older veterans with diabetes."
Researchers reviewed the EHR data of more than 275,000 veterans with diabetes who were at least 65 years old. Using predictive analytics techniques, the group identified 37 predictive factors that have been previously linked to, or are suspected to impact, mortality in older adults with diabetes.
These factors included demographic variables (age, sex, marital status); prescriptions for insulin or sulfonylureas, a class of diabetes drugs; and biomarkers like hemoglobin A1c, blood pressure, body mass index, and levels of cholesterol and triglycerides, a type of fat found in the blood. Researchers also examined inpatient and outpatient history and more than 20 medical procedures and co-occurring health disorders.
The results placed patients in three time frames for expected death: within five years, five to ten years, and more than ten years. The final predictive analytics models for five- and ten-year mortality had high predictive validity and show the importance of several individual and condition-specific characteristics that could inform clinicians and patients about life expectancy.
"We aren't saying, for example, that any of these risk factors result in decreased life expectancy," Griffith said. "However, the more risk factors that individuals have, the greater the risk of higher mortality over time."
The study’s findings could help providers use shared decision-making to establish A1c target ranges that balance treatment and risks. The models could serve as comprehensive clinical decision support tools for improved diabetes management and care.
"Our goal was to use the best available information to inform decision-making in setting glucose control targets. Doctors and patients, of course, can then use their own judgment to make a decision,” said Paul Conlin of the VA Boston Healthcare System.
The study was limited in that the analysis was based on a veteran cohort and on older adults, so the results may not generalize to other settings. However, the team stated that the predictive analytics models can be evaluated by other health systems that have similar EHR systems.
In addition, the group pointed out that it is possible that recent changes in the approach to older adults with diabetes – such as less emphasis on tight blood sugar control, as well as treatment advances for chronic kidney disease and heart failure – could impact model results.
In the future, the researchers expect that predictive analytics models will be used at the point of care to help clinicians and patients set diabetes treatment goals. The findings from this study are currently applicable to providers across all care settings, the team said.
"Our results identify multiple common conditions that can easily be identified in clinical practice and assist clinicians in shared decision-making with patients, which is a key recommendation of the VA/DoD Diabetes Guidelines," said Conlin.
VA has recently ramped up its efforts to manage diabetes among veteran populations. Earlier this year, the organization launched the Understand Your Diabetes Numbers campaign, which seeks to educate veterans and their families on hemoglobin A1c, glucose meter, blood pressure and kidney tests, and other critical measurements.
“One in four Veterans enrolled in VA healthcare services has diabetes,” said VA Secretary Robert Willkie.
“This public health campaign encourages Veterans to be proactive with their health care teams by improving their understanding of diabetes test results. The campaign reflects VA’s ongoing commitment to improve the healthcare experiences of Veterans by empowering them to be active partners in their care.”