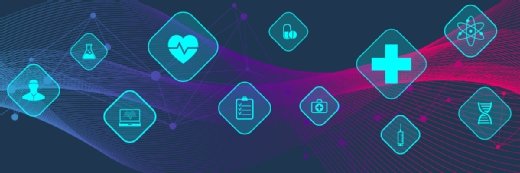
BAIVECTOR - stock.adobe.com
Penn State to Use Artificial Intelligence to Perform Health Risk Predictions
Penn State researchers aim to develop artificial intelligence algorithms to conduct risk predictions using longitudinal healthcare data, such as heart rate and blood pressure metrics.
Following the receipt of a $599,883 grant from the National Science Foundation (NSF), Penn State researchers plan to create artificial intelligence (AI)-based machine learning (ML) algorithms that can analyze longitudinal data to predict risk, including for certain healthcare conditions.
Established by Congress in 1950, the NSF is an independent federal agency that aims to enhance the progress and abilities of science. Its mission is to advance national health, along with prosperity and welfare.
The grant from the NSF will support scientists from Penn State as they work to combine ML and data to inform decision-making in healthcare.
“This grant was initially motivated by work that we were doing with healthcare data — and particularly electronic health records data,” said Vasant Honavar, PhD, professor of information sciences and technology, the Huck Chair in Biomedical Data Sciences and Artificial Intelligence, director of the Center for Artificial Intelligence Foundations and Scientific Applications, and associate director of the Institute for Computational and Data Sciences, in a press release.
“Think about every time you go to the hospital — let's say, for a routine checkup — the doctors will probably record a range of physiological parameters, for example, they may take your blood pressure, or blood sugar, or heart rate, along with the results of other tests. Basically, this is your health inventory — a bunch of parameters — that define your health status at that point in time,” continued Honavar.
This data could assist researchers in predicting health risks as well as in observing how risks change with time, according to Honavar. The researchers plan to create ML algorithms to analyze the data and glean health risk insights. Honavar also believes these algorithms could be applied to data in different fields, such as education and economics, according to the press release.
The researchers acknowledged several potential challenges, including existing gaps in data due to information not being collected daily and the large number of variables typically collected during doctor's visits. The ML algorithms that researchers plan to develop will leverage flexible modeling of longitudinal data, which may lead to more accurate predictions due to the algorithms' ability to handle large amounts of data, the press release states.
Recently, models that aim to perform risk predictions have become more common.
For example, in September, Mayo Clinic created an AI model to perform labor risk predictions. The tool collects patient data from the start of labor, as well as the patient's most recent clinical assessment and cumulative labor progress from admission. Researchers found that the labor risk score provided by the model was applicable and accurate.
Further, a study published in June found that clinical risk prediction models for sepsis, delirium and acute kidney injury achieved high performance when used in live clinical workflows within various hospitals.