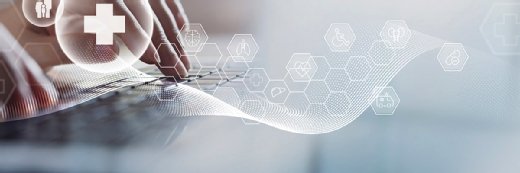
tippapatt - stock.adobe.com
Deep-Learning Algorithm Can Detect Cancer Spread Following Surgery
New research shows that a deep-learning algorithm that uses computed tomography scan images can help predict the spread of head and neck cancer outside of the neck lymph nodes.
A study conducted by ECOG-ACRIN Cancer Research Group found that using a deep-learning algorithm that leverages standard computed tomography (CT) scan images showed promise in assessing cancer spread risk among those with head and neck cancer.
Researchers noted there was a high degree of morbidity associated with standard head and neck cancer treatment. The previously conducted E3311 Phase 3 clinical trial found that low-dose radiation at 50 Gray without chemotherapy following transoral surgery provided positive results.
In this study, researchers aimed to create an AI tool that could predict cancer spread risk and thereby enable the accurate staging of the disease.
"This type of research is key since it can help identify patients with high-risk, aggressive disease and also help select appropriate patients for therapy de-escalation," said Benjamin Kann, MD, a physician at Dana-Farber Cancer Institute, assistant professor of radiation oncology at Harvard Medical School, and leader of the study for ECOG-ACRIN, in a press release.
Researchers created a neural network-based deep learning algorithm using standard CT scan images and other data from patients who participated in the E3311 trial. They used data from participants who received an assessment concluding that they had a high risk of cancer recurrence.
The researchers used factors such as tumor size, number of lymph nodes, and extranodal extension (ENE) when determining the cancer stage. In the E3311 trial, the qualifications of high-risk included an ENE exceeding 1 mm, leading patients to receive chemotherapy and high-dose radiation. Kann and his colleagues obtained trial data that showed that 311 nodes from 177 scans received annotation. Of these, 71 (23 percent) had ENE, and 39 (13 percent) had an ENE greater than 1 mm.
The tool achieved high performance in predicting ENE, which can help researchers determine the cancer stage.
“The deep-learning algorithm accurately classified 85 percent of the nodes as having ENE compared to 70 percent by the radiologists,” said Kann. “As to specificity and sensitivity, the deep-learning algorithm was 78 percent accurate versus 62 percent by the radiologists.”
Researchers intend to continue to study the algorithm's ability to improve current disease staging and risk assessment methods.
Several recent efforts have involved the use of AI in the process of treating cancer.
In September, UPMC cancer researchers began a partnership with a healthcare data analytics company Realyze Intelligence to improve treatments for early-stage breast cancer using AI and natural language understanding (NLU).
In this collaboration, UPMC is using an NLU platform from Realyze to better understand whether sentinel lymph node biopsy (SNLB) is useful in early-stage breast cancer patients under the age of 70. The platform can read unstructured clinical notes and structured data from patient EHRs. The data was then used to create a breast cancer model to identify lymph node positivity.
Another clinical trial involved researchers from the Artificial Intelligence in Medicine Program of Brigham and Women’s Hospital creating a deep-learning algorithm to improve lung cancer radiation therapy treatment. The model was designed to detect non-small cell lung cancer tumors within CT scans. The goal of the program was to improve treatment methods and pave the way for the future use of AI in medicine.