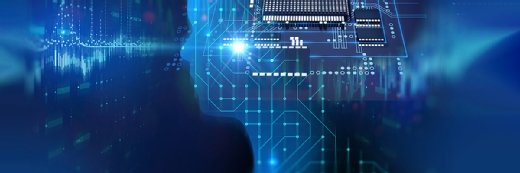
Getty Images
ML Tools Facilitate Early Detection of Autism Spectrum Disorder
Researchers at Penn State have found that a machine-learning model using medical claims data can predict the risk of autism spectrum disorder in young children.
A new study published in BMJ Health & Care Informatics shows that machine-learning (ML) models can accurately predict autism spectrum disorder (ASD) risk in children 18 to 30 months old using health claims data.
According to the Centers for Disease Control and Prevention, ASD is a developmental disorder caused by differences in the brain. Some of these differences are known, such as a genetic condition, but many causes are yet unknown. Because these differences are often unknown, diagnosing ASD can be difficult.
However, early intervention has been shown to significantly improve a child’s development and quality of life. ASD screening tools currently exist but have shown insufficient accuracy, the study notes.
To facilitate early detection and intervention, the researchers sought to create accurate ML-based tools that could predict the risk of ASD using medical claims data.
To create their tools, the researchers identified 12,743 children with ASD and a random sample of 25,833 children without ASD using the MarketScan Health Claims Database 2005–2016. From these data, the team developed logistic regression and random forest models designed to predict ASD diagnoses between 18 and 30 months of age using demographics, medical diagnoses, and healthcare service procedures as predictors.
Overall, the logistic regression and random forest models achieved an area under the receiver operating characteristic curve (AUROC) of 0.758 and 0.775, respectively, for predicting ASD diagnosis at 24 months. The random forest model for prediction at 24 months improved significantly when predictor variables were separated by outpatient and inpatient visits, achieving an AUROC of 0.834, with 96.4 percent specificity and 20.5 percent positive predictive value at 40 percent sensitivity.
The study authors concluded that the findings demonstrate the feasibility of using an ML-based tool for ASD prediction in young children, noting that such a tool has the potential for monitoring ASD risk in the general pediatric population and early detection of high-risk children for targeted screening.
This is not the first time artificial intelligence (AI) and ML have been used to bolster ASD detection and screening.
In May, research published in npj Digital Medicine showed that an AI-based medical device could assist clinicians in primary care settings accurately diagnose ASD in children up to 6 years old.
In April last year, researchers found that an ML-based mobile app that analyzed toddlers’ eye movements while watching videos could distinguish children diagnosed with ASD.
Earlier in 2021, researchers identified blood- and maternal autoantibody-based biomarkers indicating the likelihood and severity of ASD using machine-learning tools.