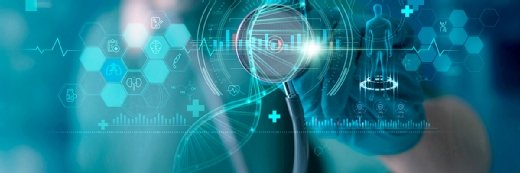
ipopba/istock via Getty Images
Artificial Intelligence Model Can Support Rare Disease Diagnoses, Treatment
New research shows how an artificial intelligence model can function as a search engine for databases to support the diagnosis and treatment of various rare conditions.
Investigators from the Mahmood Lab at Harvard Medical School and Brigham and Women’s Hospital found that an artificial intelligence (AI) model, known as Self-Supervised Image Search for Histology or SISH, displayed the ability to improve the diagnosis and treatment processes for rare conditions.
Diagnosing a rare disease and determining the best treatment route to take can be very difficult, according to the press release.
To ease these processes, the researchers developed SISH, a deep-learning algorithm that can learn image features to detect similar cases within large pathology image repositories. Researchers described SISH as a search engine for pathology images with various applications, including helping identify rare conditions and potential treatment actions.
Researchers tested the abilities of SISH to gather interpretable disease subtype information for cancers. They found that the tool was able to successfully obtain information from a database consisting of tens of thousands of whole slide images, representing over 22,000 patient cases.
Further, the speed of image retrieval of the algorithm outperformed other methods in several cases, including disease subtype retrieval.
“We show that our system can assist with the diagnosis of rare diseases and find cases with similar morphologic patterns without the need for manual annotations and large datasets for supervised training,” said senior study author Faisal Mahmood, assistant professor of pathology at HMS at Brigham and Women’s, in the press release. “This system has the potential to improve pathology training, disease subtyping, tumor identification, and rare morphology identification.”
Despite these positive results, researchers noted several limitations of the algorithm, including a lack of context awareness in large tissue slides and the use of a single imaging modality.
However, researchers remained optimistic about the capabilities of the model.
“As the sizes of image databases continue to grow, we hope that SISH will be useful in making identification of diseases easier,” said Mahmood. “We believe one important future direction in this area is multimodal case retrieval, which involves jointly using pathology, radiology, and genomic and electronic medical record data to find similar patient cases.”
In the past, various AI models have assisted physicians in diagnosing and assessing risk for several medical conditions.
A Mayo Clinic study from September found that an AI-based screening strategy could assist in detecting atrial fibrillation. Researchers noted that the AI system could use electrocardiogram data to detect the disease, recognizing high-risk patients that are often overlooked.
Another study from September described how creating an AI-based risk prediction model could assist in determining potential child delivery outcomes. Researchers found that the model presented accurate labor risk scores by gathering patient data from the start of labor, the most recent clinical assessment, and cumulative labor progress from admission.