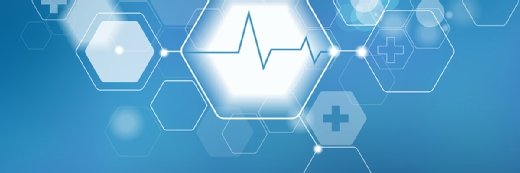
AlfaOlga/istock via Getty Images
New Patient Outcome Prediction Tool May Improve Clinical Decision-Making
University of Florida researchers have developed a predictive analytics tool that can identify patients at risk of poor outcomes, which may improve the accuracy and speed of clinical decision-making.
A new study published last week in PLOS Digital Health shows that an artificial intelligence (AI)-based predictive analytics tool can accurately forecast patient risk for deterioration and other adverse outcomes within six hours of hospitalization.
According to data from the US Department of Health and Human Services (HHS) Office of Inspector General released in May, 12 percent of Medicare patients experienced adverse events during their hospitalizations in October 2018. These events led to longer hospital stays, permanent harm, or death, and often required life-saving interventions.
The OIG report shared multiple recommendations for the Agency for Healthcare Research and Quality (AHRQ) and the Centers for Medicare and Medicaid Services (CMS). Among these was the recommendation to improve data capture and surveillance to prevent adverse events in hospitals.
To support early intervention and improve outcomes in the hospital setting, University of Florida (UF) researchers developed a tool to provide more timely, accurate insights into patients’ conditions and likely medical outcomes.
The research team created an EHR dataset containing data on 75,762 adult patient admissions to a tertiary care center that lasted six hours or more from 2014 to 2016. These data were then used to train a predictive analytics algorithm to leverage six routinely collected vital signs — systolic and diastolic blood pressure, heart rate, respiratory rate, temperature, and oxygen saturation — measured within the first six hours of hospitalization. Using the vital sign measurements, the algorithm grouped patients based on disease phenotypes and physiological signatures.
The tool divided patients into four groups, labeled Physiotypes A through D. Those in Physiotype A exhibited early and persistent hypotension, B experienced low-grade inflammation, and C showed increased heart activity. These conditions can be severe in their early stages but have the potential to be resolved and result in favorable patient outcomes, according to the press release.
Physiotype D patients exhibited the most significant prevalence of chronic cardiovascular and kidney disease, and these patients were more likely than the others to die within three years of hospitalization.
Each of the conditions discussed above can lead to adverse outcomes, but the predictive analytics tool collects and analyzes patient data to give clinicians a better picture of the patient's health and improve clinical decision-making, according to the researchers.
“It’s really like an early warning sign. Within six hours, it can help identify patients who may be at risk of not having a good outcome. It tells us which patients may be at risk of deteriorating and who needs more attention right away,” said Azra Bihorac, MD, senior associate dean for research affairs at the UF College of Medicine and director of UF’s Intelligent Critical Care Center, in the press release.
Other health systems are also leveraging analytics to tackle adverse events and improve outcomes.
In a recent interview with HealthITAnalytics, Santiago Borasino, MD, medical director of the cardiovascular intensive care unit at Children’s of Alabama, shared how the hospital is using real-time analytics to address patient deterioration, assess extubation readiness, and conduct code event reviews as part of its ICU Liberation strategy.