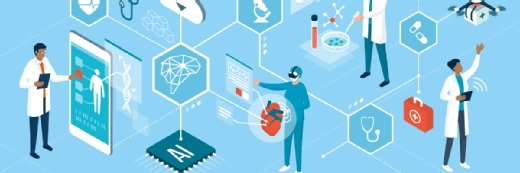
Getty Images
Researchers Develop Machine-Learning System to Detect Public Health Threats
Researchers have developed an automated machine-learning system that can provide 'pre-syndromic' public health surveillance to identify new or rare disease clusters.
Researchers from New York University’s Machine Learning for Good Laboratory (ML4G Lab), Carnegie Mellon University, and the New York City Department of Health and Mental Hygiene (NYC DOHMH) have developed an automated machine-learning system designed to detect rare or previously unseen disease clusters.
According to the press release shared with HealthITAnalytics via email, current automated systems used to identify public health threats rely on “syndromic surveillance” to detect existing threats but can fall short of identifying new ones.
“Existing systems are good at detecting outbreaks of diseases that we already know about and are actively looking for, like flu or COVID,” said NYU Professor Daniel B. Neill, PhD, director of the ML4G Lab, in the press release. “But what happens when something new and scary comes along? Pre-syndromic surveillance provides a safety net to identify emerging threats that other systems would fail to detect.”
To close this surveillance gap, the researchers designed a system that would enable public health officials to respond more quickly and effectively to emerging unusual or novel threats. To develop the system, known as Multidimensional Semantic Scan (MUSES), the research team relied on a “pre-syndromic” surveillance approach, which leverages digitally communicated data on patient conditions.
Current surveillance systems, in contrast, rely on classifying case data under existing disease syndromes. By not automatically classifying cases this way, a “pre-syndromic” system can support the rapid detection of newly emerging diseases or conditions that public health authorities are unaware of.
To create the system, the researchers used anonymized chief complaint data from hospital emergency department (ED) visits. These complaints are typically provided by the patient in their own words and recorded by an ED triage nurse or clinician, the press release states.
The system then uses these complaints to identify trends and patterns in the words or phrases used by patients, enabling the potential detection of a localized case cluster. The system can also incorporate feedback from public health practitioners to help distinguish between relevant and irrelevant clusters, reduce false positives, and provide decision support to health departments and hospitals.
The press release further notes that MUSES offers significant surveillance advances to public health institutions and hospitals because it eliminates the need for pre-defined syndrome categories, identifies case clusters using multi-dimensional scan statistics to enable the detection of emerging threats that may impact certain spatial areas or patient groups, and uses a “practitioner in the loop” approach to focus on relevant patterns and provide actionable insights.
Other approaches to public health surveillance leveraging artificial intelligence (AI) have also been proposed recently.
Last year, researchers unveiled an early-warning system that uses predictive analytics to optimize infectious disease surveillance in the wake of the COVID-19 pandemic. The system was designed to provide a cost-effective early-warning system to enable quick response to outbreaks and prevent the further spread of influenza in locations without existing surveillance. It relies on data streams from various other locations, which are informed by human movement.