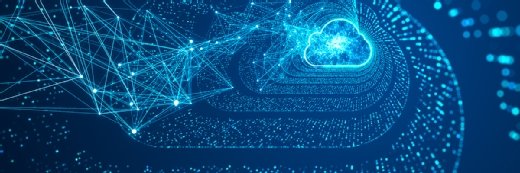
Getty Images/iStockphoto
Researchers Suggest Changes to FDA Oversight of AI Breast Cancer Screening
New commentary highlights questions around the accuracy, appropriate use, and clinical utility of AI for breast cancer screening and suggests ways to improve current regulatory approaches.
In a special communication published this week in JAMA Internal Medicine, Yale researchers explore the current regulatory processes for artificial intelligence (AI)-based breast cancer screening tools, sharing the limitations, advantages, and potential recommendations for improvement in US Food and Drug Administration (FDA) regulatory approaches.
The researchers began by describing the current FDA regulatory process for AI tools, which is centered around the Software as a Medical Device (SaMD) standard. SaMD is defined as "software intended to be used for one or more medical purposes that perform these purposes without being part of a hardware medical device."
Products classified as SaMD are currently reviewed through three FDA medical device pathways: 510(k), De Novo, and Premarket Approval (PMA). The pathway chosen for a review depends on the risk associated with a device and whether there is a similar FDA-approved or-cleared device that already exists.
The FDA has also proposed a voluntary program, the Software Pre-Cert Pilot Program (Pre-Cert program), designed to address the challenges of regulating SaMD, including AI-specific challenges like adaptive algorithms.
Further, the researchers discussed the evidence used to support FDA clearance and approval of AI products indicated for breast cancer screening and the advantages and limitations of current regulatory approaches.
They found that nine AI products for breast cancer screening that had been cleared or approved by the FDA relied mainly on sensitivity, specificity, and area under the curve as performance outcomes and on tissue biopsy as the criterion for breast cancer screening accuracy.
Though the evidence was used to support FDA clearance or approval, it also highlights gaps and advantages in the current approval process, the researchers posited. One advantage, they noted, is that most FDA-approved AI products for breast cancer screening use reported test accuracy for identifying breast cancer as the key metric for demonstrating substantial equivalence between a new device and one that is already FDA approved or cleared, which is a requirement for 510(k) review.
However, some approaches to demonstrate substantial equivalence also have several weaknesses, including increased risk of bias, limited generalizability, and the notion that focusing on cancer detection does not necessarily translate to improved health because of false-positive results and overdiagnosis.
To combat these shortcomings, the researchers recommended that the FDA strengthen its evidentiary standards for AI product clearance. To do so, the research team suggests that the agency include specific requirements for study design, outcomes, study populations, and validation approaches while also modifying its voluntary guidance, to which AI product manufacturers are strongly incentivized, but not required, to adhere.
Further, the researchers recommended that the FDA strengthen requirements for and reporting of study design features, such as clinical diversity and generalizability. They also noted that a postmarketing surveillance system is needed alongside these measures to help detect unintended consequences of AI when applied by physicians, deviations in performance compared to the findings of controlled studies, or changes in intended use.
The authors concluded that increased FDA evidentiary regulatory standards, development of improved postmarketing surveillance and trials, a focus on clinically meaningful outcomes, and engagement of key stakeholders could help ensure that AI tools support improved breast cancer screening outcomes.
This commentary comes as the FDA continues to work toward solidifying regulations for AI and machine learning (ML)-based health tools.
In September, the FDA shared new guidance recommending that some AI tools be regulated as medical devices as part of the agency’s oversight of clinical decision support (CDS) software. The new guidance includes a list of AI tools that should be regulated as medical devices, including devices to predict sepsis, identify patient deterioration, forecast heart failure hospitalizations, and flag patients who may be addicted to opioids.