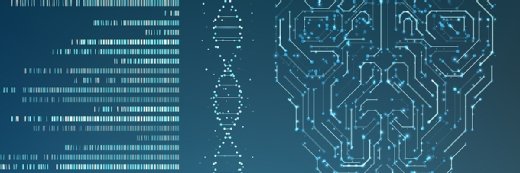
Nobi_Prizue/istock via Getty Ima
AI Can Help Improve Identification of High-Cost Health Plan Members
New research shows that a risk prediction model that uses artificial intelligence and social determinants of health data can help identify high-cost health plan members.
A recent study published in the American Journal of Managed Care found that identifying high-cost members was made easier through the implementation of artificial intelligence (AI) and the analysis of patient demographics.
Identifying high-cost members is essential for payers and providers, as it offers them information on preventing excessive spending. Traditionally, payers and care delivery organizations rely on care management efforts to reduce medical expenditure; however, this can often be challenging due to the limitations of incorporating other data sources, according to the study authors.
In the study, researchers aimed to implement a risk prediction model that uses AI to analyze information such as claims data, demographics, social determinants of health (SDOH) data, and admission, discharge, and transfer alerts (ADT) to better identify high-cost members.
Researchers used data from a Medicaid accountable care organization (ACO) gathered from 61,850 members enrolled between May 2018 and April 2019.
Researchers then estimated risk scores for each member using two separate models. The first model was developed by Medical Home Network and relied on AI to analyze data related to SDOH, and activity related to ADT, along with claims and demographic characteristics. However, the second model, known as the Chronic Illness and Disability Payment System (CDPS) , only used demographic and claims information.
When comparing the success of the two models, researchers considered the members with the highest 5 percent of AI risk scores and members with the highest 5 percent of CDPS risk scores, and calculated the mean, median, and total spending for the members.
Initially, the study noted that among members with the longest claims history, the top 5 percent of members by spending incurred a total of $38.5 million. Among the top 5 percent of AI risk scores, the total cost incurred was $20.8 million, with a mean of $14,349 per member and a median of $7,265 per member. Among the top 5 percent of CDPS scores, the mean and median spending were $11,808 and $3,753, respectively.
Based on this information, the researchers found that the AI model could perform a more accurate analysis of the highest-risk members and their spending. In addition, they found that those the AI model identified as high-risk had higher spending than those identified by the CDPS model.
Despite these conclusions, researchers noted a few limitations, mainly related to the data coming from a single ACO from a single geographic area and a single 12-month period.
Various studies have provided insight into how AI can support healthcare processes.
For example, a study published in February discussed how AI-based scheduling led to lower levels of physician burnout. After assessing data noted over several years, researchers emerged with a prominent statistic: AI led to a significant drop in time spent on scheduling.
They also found that it led to higher engagement from physicians, along with improved work-life balance.
Further, AI is increasingly being used in diagnostic processes.
Another study published in April found that AI led to improved heart disease diagnoses. After testing out a new AI system on thousands of ultrasound images and engaging it in an extra round of deep learning, researchers determined that the model ultimately led to more accurate diagnoses.