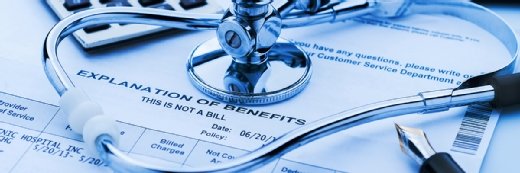
Christian Delbert - stock.adobe.
Predictive Analytics Use EHR Data for Hospital Readmissions
Researchers have developed a suite of predictive analytics tools to reliably identify all-cause 30-day pediatric readmission risk before hospital discharge.
In a new study published last week in JAMA Network Open, researchers found that a suite of predictive analytics tools leveraging readily available EHR data can accurately identify all-cause 30-day readmission risk for pediatric patients prior to hospital discharge.
The study authors noted that despite the fact that hospital readmission is widely used as a quality care measure and that models to predict adult readmission exist, there are no such tools to predict risk of pediatric hospital readmission.
To address this gap, researchers set out to develop and validate a tool identifying pediatric patients before hospital discharge who are at risk for all-cause 30-day subsequent readmission. To do so, they leveraged EHR-derived data from admissions at Ann & Robert H. Lurie Children’s Hospital of Chicago (LCH) from January 1, 2016, to December 31, 2019.
To model all-cause 30-day readmission, researchers relied on three years of LCH discharge data. Ongoing length of stay (LOS), use of specific therapies, and past utilization were used as predictive factors for all-cause 30-day readmission.
From this process, the research team derived three models: a recent admission model (RAM), which included children 6 months or older with 1 or more prior hospitalizations within the last 6 months; a new admission model (NAM), which included children 6 months or older with no prior hospitalizations in the last 6 months; and a young infant model (YIM), which included children younger than 6 months.
The models were designed to act as a complementary suite for pediatric readmission risk prediction and were validated with an additional year of discharges outside of those used in the training set.
Overall, the models analyzed 29,988 patients with 48,019 hospitalizations. Of these admissions, 50.1 percent were for children younger than 5 years. The number of patients who were readmitted within 30 days varied by cohort. Approximately 36.2 percent in the RAM cohort, 7.4 percent in the NAM cohort, and 12.2 percent in the YIM cohort were followed within 30 days by a readmission.
In the RAM cohort, prior utilization, current or prior procedures indicative of severity of illness, such as transfusion, ventilation, or central venous catheter, commercial insurance, and prolonged LOS were associated with readmission. In the NAM cohort, procedures, prolonged LOS, and emergency department visits in the past six months were associated with readmission. In the YIM cohort, LOS, prior visits, and critical procedures were associated with readmission.
The performance of the risk models ranged from acceptable to excellent. The area under the receiver operating characteristics curve was 83.1 for the RAM cohort, 76.1 for the NAM cohort, and 80.3 for the YIM cohort.
These findings, researchers concluded, indicate that such risk prediction models may improve discharge preparedness and prevent high-risk readmissions in the future, but further research is needed.
This research is part of an ongoing interest in applying risk stratification and predictive analytics to pediatrics.
In a 2020 interview with HealthITAnalytics, Gertrude Leidich, vice president of quality and medical staff services at Texas Children’s Hospital, and Rahul Shah, MD, vice president and chief quality and safety officer at Children’s National Health System, shared how payers and providers can integrate data to improve pediatric risk scoring and efficiently allocate clinical and financial resources.
Leidich noted that siloed pediatric data severely limits providers’ abilities to accurately understand a patient’s risk for hospitalization or recovery. This lack of centralized, risk-adjusted data and methodology for pediatrics can also make internal and external comparisons nearly impossible. To combat this, Texas Children’s and Children’s National teamed up with Configo Health, Inc to integrate multiple pediatric data sets, accurately establish standardized risk scores, and improve outcomes.