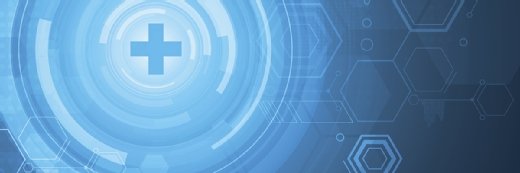
traffic_analyzer/DigitalVision V
Researchers Outline Strategies to Develop High-Quality Medical ML Models
Researchers have shared practical techniques to develop robust artificial intelligence and machine-learning models for clinical research and medicine.
A study published this month in BMC Medical Research Methodology outlines how to develop high-quality machine learning (ML)-based models for use in clinical research and medicine through the use of practical techniques such as data pre-processing, hyperparameter tuning, and model comparison.
To provide these guidelines, the researchers trained and validated multiple ML models to demonstrate best practices. The models were designed to classify breast masses as benign or malignant using mammography image features and patient age. Model predictions were compared to histopathologic evaluations of the same breast images to measure performance.
Using this example, the researchers provided step-by-step instructions on performing an ML analysis, starting with data preparation and ending with model evaluation. They also utilized open-source software and data to allow others to practice the techniques outlined in the paper, which is part of a series on ML in medicine.
The researchers begin by discussing data pre-processing, which consists of data cleaning and feature engineering. Data cleaning refers to the process by which incorrect, irrelevant, and duplicate data are removed and missing data are addressed.
The authors noted that addressing missing data requires substantial knowledge of the data, including the context in which it was collected and the context in which the ML model will be used. For this reason, they recommend multidisciplinary collaboration between clinicians and data scientists to adequately clean the data.
Feature engineering describes the statistical approaches used to prepare data so that the ML model can utilize them more effectively. Examples include data normalization, transformation, feature selection, dimensionality reduction, and data type conversion.
The next step in the process is hyperparameter tuning. Hyperparameters control the configuration of a particular ML algorithm. They can be classified into optimization hyperparameters and model hyperparameters.
Optimization hyperparameters are designed to control the training process and learning rate of a given model, while model hyperparameters specify an algorithm’s architecture, such as the number of layers in a neural network.
The researchers also noted that hyperparameters are distinct from model parameters. Model parameters are directly derived from data during the training process, they explained. Hyperparameters, in contrast, are pre-specified manually and can vary across different models.
Because of these specifications, hyperparameters are critical to ML model performance for a given task on a particular dataset. The process of identifying the optimal combination of hyperparameters for a particular model is known as hyperparameter tuning or optimization.
Finally, the researchers highlighted the importance of model comparison. Using statistical tests, different models can be compared to evaluate model performance and determine if differences in model performance are statistically significant.
They noted that while model performance is crucial, researchers may not always want to choose the model with the best performance on the testing dataset. Other factors, such as model generalizability and ease of implementation, are also key to developing a high-quality ML tool. For instance, they described an example in which scientists would choose the simplest model with a certain degree of performance from the best-performing model to prioritize these factors.
The authors concluded that following these guidelines may help to improve model generalizability and reproducibility, which may, in turn, help bolster trust in medical ML applications.