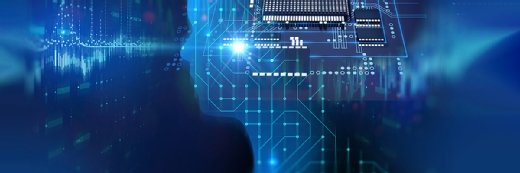
Getty Images
Predictive Analytics-Based Disease Management Outreach Reduces Spending
New research shows that a predictive analytics-based disease management outreach program effectively reduced medical spending among chronic heart failure patients by 20 percent.
A study recently published in the American Journal of Managed Care found that compared with a non-predictive algorithm-driven disease management (DM) program, a disease management outreach program supported by predictive analytics effectively reduced medical spending among patients with chronic heart failure (CHF).
According to the Centers for Disease Control and Prevention (CDC), about 6.2 million adults in the US have heart failure, a condition that appeared on 379,800 death certificates in 2018. The CDC also noted that heart failure cost the nation an estimated $30.7 billion in 2012.
In this study, researchers aimed to determine how the capabilities of a predictive algorithm-driven DM outreach program compared to a non-predictive algorithm-driven DM program, using healthcare spending and utilization as measures.
The study population consisted of Medicare Advantage members with CHF. All information used in the study came from claims data from 2013 to 2018. Considering data such as demographics, health risk, and health plan information regarding age, gender, and a standard concurrent risk score, known as a Verisk score, researchers identified which CHF patients were at a higher risk for hospitalization using a predictive algorithm, followed by DM outreach to those with this higher risk.
Researchers noted that the intervention group was matched with members with similar concurrent medical risk profiles who received similar DM outreach through the standard process of the insurer, which did not involve the use of the predictive algorithm.
There were 1,592 intervention group members matched to 1,592 members in the standard care group. The mean age of the study population was about 80 years, 55 percent were women, and a large portion had pharmacy benefits. The study sample's mean Verisk risk score, which measures the present health risk of the member, was about 31. But the predictive model risk score was higher among the predictive outreach group and low among the standard outreach group.
According to regression analyses, in the year after the outreach, those who were at a high risk of hospitalization in the intervention group had a 6 percent lower chance of hospitalization and a 6 percent lower probability of emergency department visits than the standard care group. This also led to a 20 percent decrease in total outpatient spending.
Based on this, researchers concluded that using the prediction-driven DM outreach program among patients with CHF effectively reduced medical spending within a year following the intervention.
Predictive analytics is increasingly being applied to patient care. A study published in JAMA Network Open in November found that a set of predictive analytics tools can leverage EHR data to identify 30-day readmission risk among pediatric patients.
Researchers noted that previously, tools for predicting the risk of pediatric hospital readmission did not exist. To address this, they gathered EHR data from a single hospital location and considered three different predictive factors to assess pediatric readmission risk. The factors were the ongoing length of stay, use of specific therapies, and past utilization.
Researchers then developed three models for risk prediction and validation. The models' performances ranged from acceptable to excellent.