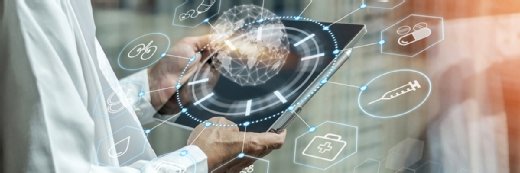
Chinnapong - stock.adobe.com
Researchers to Develop Machine-Learning System to Automate Insulin Delivery
A $1.2 million NIH grant is supporting the development of a machine-learning tool to enhance the accuracy of an artificial pancreas system for type 1 diabetes patients.
Researchers from the Illinois Institute of Technology (IIT) have received a $1.2 million grant from the National Institutes of Health (NIH) to develop a machine-learning (ML) tool for automated insulin delivery as part of a larger project to build an artificial pancreas system for patients with diabetes.
According to the press release, the average patient with type 1 diabetes must make between 100 and 200 decisions daily to keep their glucose levels stable. If this person forgets or misjudges the appropriate insulin dose, they can experience moderate to severe symptoms because of high or low blood sugar. Further, individuals whose glucose levels are often outside their target range or are regularly too high can experience serious long-term complications.
To help patients better manage their blood glucose, the research team developed a multivariable artificial pancreas system in 2020. The system consists of a glucose sensor, a wristband, a dedicated smartphone, and a fully automated insulin pump that doesn’t require manual inputs from the user. With these, the artificial pancreas can automatically monitor and infuse insulin according to metabolic changes, like those that occur in response to food consumption and physical activities.
The new NIH-funded project takes this system further by leveraging ML to analyze a patient’s past behavior and personalize the tool’s decision-making algorithm to improve its ability to determine whether someone is or soon will be engaging in a behavior that could impact their glucose levels, the press release states.
According to the researchers, this predictive ability is key because there is a delay between when the insulin is administered and when it begins to take effect.
“If someone eats lunch at noon every day and the meal has usually 20 to 30 grams of carbohydrates, then if their current blood glucose level is not very low, at 11:45 we could say, ‘Everything indicates that the trend of this day is a typical weekday for this person, so let’s give them, not the whole dose of insulin, but a little bit of it so that it will blunt the meal effect on the glucose,’” said Ali Cinar, PhD, professor of chemical engineering and lead on the project, in the press release.
Using predictive analytics, the system will match the pattern of the current day to an individual’s known behavior patterns and assign a probability to the likelihood that they will engage in a glucose-impacting behavior, such as eating lunch. If the patient is likely to eat lunch, the system will administer some insulin and continue to monitor glucose levels. If glucose levels start to rise because the patient is eating lunch as predicted, the system will administer an appropriate amount of additional insulin.
The system is also designed to be more streamlined and easier to use than existing tools, the press release notes. Currently, automated insulin delivery systems require manual user inputs, such as carbohydrate calculations and exercise adjustments, which are prone to human error.
These systems can also miss more complex factors and relationships that can impact glucose levels simultaneously, such as a combination of stress, exercise, and food, according to the press release.
The artificial pancreas system aims to incorporate these factors into automatic decision-making. In the future, if the system has access to enough historical data on a person, it could potentially learn to predict their behavior, even if they have seemingly irregular habits, Cinar concluded.
This is the latest work that uses ML to bolster diabetes management.
In 2020, researchers from Oregon Health & Science University (OHSU) developed an ML-based clinical decision support system to help patients control their glucose levels and enhance type 1 diabetes management. The tool’s algorithm was trained to identify causes of hypo- and hyperglycemia and determine necessary insulin adjustments from 12 potential recommendations. The system then provided insulin injection recommendations for each patient, which were shown to be in agreement with clinicians 67.9 percent of the time.