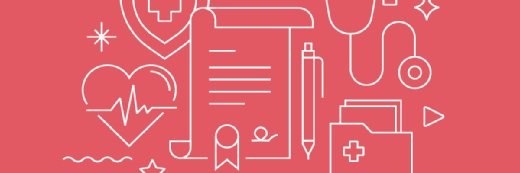
cnythzl/DigitalVision Vectors vi
Machine-Learning Models Predict 6-Month Ovarian Cancer Mortality
A new study shows that an ensemble of machine-learning models can predict six-month mortality for patients with ovarian cancer using patient-reported outcome data.
A study published this month in Scientific Reports found that an ensemble of machine-learning (ML) models can accurately predict six-month ovarian cancer mortality using patient-reported outcome data, like symptom severity.
According to the American Cancer Society (ACS), ovarian cancer accounts for more deaths than any other cancer of the female reproductive system, ranking fifth in cancer deaths among women overall. The ACS further notes that only about 20 percent of ovarian cancers are identified at an early stage. Five-year survival rates for ovarian cancer vary based on cancer type and stage, averaging from 49 to 93 percent based on data collected between 2011 and 2017.
Some research indicates that only 29 percent of those diagnosed with late-stage ovarian cancer survive five years after diagnosis, according to the study.
The study additionally notes that the small number of cancer cases detected at an early stage may be a result of ineffective screening.
With recurrent or late-stage disease, treatments to maximize survival and reduce symptoms eventually come at the expense of patient quality of life, with some treatments being associated with significant side effects like neuropathy, mouth sores, nausea, vomiting, and fatigue, the researchers state.
The research team further highlighted that many patients with recurrent ovarian cancer receive aggressive care end-of-life, which some clinicians and healthcare organizations have argued may represent a quality-of-care issue.
Along with these concerns, the study states that oncologists tend to overestimate survival and patient prognoses, which can lead to the overuse of chemotherapy or other treatments and underutilization of hospice and palliative care services.
Not only that, but accurately estimating ovarian cancer mortality can inform clinical decision-making and improve end-of-life care, the researchers note. Thus, they set out to develop a prediction tool that can reliably identify when an ovarian cancer patient is reaching the end of their life.
They began by recruiting 243 patients from a single large academic cancer center in the US and collecting baseline demographic data after study enrollment. Patient-reported outcome measures (PROM) assessments were administered at baseline and then every 90 days until discharge to hospice or death. The assessments measured symptom severity and interference, depression, anxiety, health-related quality of life, and cancer-related quality of life, for a total of 1,319 assessments.
Using these data, the researchers trained seven ML algorithms to predict death within 180 days of a PROM assessment, a timeframe they stated is suitable for signaling a transition to the end of life and prompting productive end-of-life conversations.
The research team combined the predictions from these models into a final classification from which mortality predictions could be derived. Each algorithm’s accuracy, sensitivity, specificity, and area under the receiver operating characteristic curve (AUROC) was assessed to evaluate model performance.
Overall, the ensemble of models correctly identified 25 of the 35 women in the testing dataset who died within 180 days of PROM assessment, achieving an accuracy of 0.79, and a sensitivity, specificity, and AUROC of 0.71, 0.80, and 0.76, respectively.
These findings indicate that the model could be used to drive data-driven end-of-life care and address current shortcomings in care delivery, thereby underscoring the potential of leveraging PROM in oncology prediction modeling, the researchers stated.
This research is the latest in a string of studies assessing the use of ML for cancer mortality prediction.
In May, researchers validated and developed a model capable of predicting six-month mortality for patients with advanced solid tumors who are considering a new line of therapy (LoT) using EHR data from inpatient or outpatient University of Utah Health and Huntsman Cancer Institute (HCI) care settings alongside HCI hospital-based cancer registry (HCI-CR) information.
The researchers developed their model using 111 relevant predictive features from cancer prognostication literature and created two additional limited models using 45 and 23 features. The models all achieved high performance, with 66 percent of those flagged by the models as having a low chance of survival dying within six months.