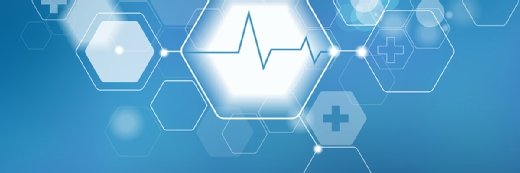
AlfaOlga/istock via Getty Images
Using Social Determinants of Health Data in Machine Learning Reduced Bias
A recent study found that adding components related to social determinants of health to machine learning led to lower bias levels.
Published in Circulation: Heart Failure, a recent study conducted by Northwestern Medicine researchers found that adding social determinants of health (SDOH) data into machine learning models led to less bias when predicting outcomes for heart failure patients.
Healthcare bias can lead to detrimental outcomes for patients. For example, in April, a study published in PLOS digital health found that digital health biases and data gaps involved in the production of artificial intelligence (AI) tools can lead to healthcare disparities.
But Northwestern Medicine researchers have found that SDOH data can help curb bias, thereby reducing disparities.
The study included performance reviews of five machine-learning models often used to determine the outcomes of hospitalized patients. They then integrated 15 measures of SDOH, all of which were provided by the American Heart Association, into these machine-learning models. These measures included Social Deprivation Index scores and Area Deprivation Index scores.
Prior to adding SDOH data, the best-performing model underdiagnosed several underserved populations, including female, Black, and socioeconomically underserved patients, the researchers noted.
However, the addition of SDOH data led to improvements in the fairness of the models.
“We show that for minority populations, the machine learning models actually performed worse than for white individuals. We also show that for people with poor socioeconomic status, let’s say for those uninsured or for people that have Medicaid, the model also performed worse and missed people that are at a higher risk of dying or have a higher risk of staying in the hospital longer,” said senior author of the study Yuan Luo, PhD, associate professor of preventive medicine and pediatrics, and chief AI officer at the Northwestern Clinical and Translational Sciences (NUCATS) Institute and the Institute for Augmented Intelligence in Medicine, in a press release.
Researchers also noted that these findings provide a framework for other researchers to develop predictive models that can mitigate bias and help reduce healthcare disparities.
“Our purpose is to also let people know that you can actually correct these models by adding those social determinants of health variables, making models explicitly aware of such variables so that they can learn to correct for those biases,” said Luo.
These study findings come as AI use in healthcare increases alongside growing concerns regarding bias in AI models.
The Institute for Augmented Intelligence in Medicine at Northwestern Medicine Feinberg School of Medicine created the Center for Collaborative AI in Healthcare in December.
With goals related to advancing AI science, engineering, and translation across healthcare specialties, this center aimed to serve as a hub for research, training, and collaboration. Through this new center, researchers will develop comprehensive AI and machine-learning models to support the integration of multiple healthcare data modalities, as well as create datasets to integrate phenotypic and multi-omic data modalities, supply educational opportunities, and support the integration of data assets from different Feinberg centers.
But a report published in August highlighted the practices that can lead to bias in the AI and machine-learning models increasingly used in radiology.
According to the report, 12 suboptimal practices occur during the data handling process that can potentially lead to bias. But some steps can be taken to mitigate these biases, like using multiple datasets and engaging in knowledge sharing with clinical and data science experts.