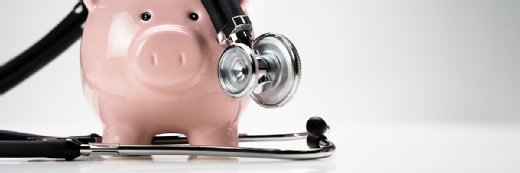
AndreyPopov/istock via Getty Ima
Researchers Assess ML Algorithm for Undiagnosed Dementia Detection
Indiana-and Florida-based researchers are conducting research trials to test the ability of a machine-learning algorithm that uses EHR data to determine early signs of dementia.
A group of researchers is conducting the Digital Detection of Dementia study that will evaluate the use of an artificial intelligence (AI)-based machine-learning (ML) algorithm that uses natural language processing and EHR data to identify undiagnosed Alzheimer’s disease and related dementias.
The researchers are from the Regenstrief Institute, Indiana University–Purdue University Indianapolis (IUPUI), and the medical schools of Indiana University and the University of Miami.
According to the Centers for Disease Control and Prevention (CDC), about 5.8 million people in the US have Alzheimer's disease and related dementias.
In addition, many cases of the disease go unrecognized and, therefore, undiagnosed, according to a press release.
Further, not many primary care practices can detect Alzheimer's disease in a timely manner. Regenstrief Institute and Indiana University School of Medicine faculty member Malaz Boustani, MD, noted that the little time that primary care clinicians have with patients, the need to focus on other issues, and the stigma surrounding dementia are some reasons why the condition often goes undiagnosed.
“Between 50 to 80 percent of dementia cases are unrecognized by the healthcare system in the U.S. And, if you include patients living with mild cognitive impairment, that number might actually climb to higher than 80 percent of cases that are not recognized,” said Boustani, in a press release.
To address this issue, the scientists created an ML algorithm. Known as a passive digital marker, the tool uses natural language processing to obtain unstructured information along with structured data from the patient's EHR.
“The benefit of this approach is that the information has already been collected and requires no extra effort from the patient or the provider," said Zina Ben Miled, PhD, a Regenstrief Institute-affiliated scientist and an IUPUI associate professor of electrical and computer engineering, in a press release. "Through machine learning algorithms and natural language processing, we can use this data to identify people who may be at risk for Alzheimer’s disease without the need for invasive and costly tests.”
Miled developed the passive digital marker tool along with Boustani.
The Digital Detection of Dementia study will assess the efficacy of the passive digital marker tool. It includes a clinical trial that has already started in Indianapolis and another clinical trial slated to begin in early 2023 in Miami.
The clinical trials are similar in structure and comprise three parts: a usual primary care approach, an approach involving the passive digital marker that uses AI, and an approach involving the novel passive digital marker along with a specially designed patient-reported outcomes survey.
All patients, regardless of which arm they participate in, will be followed for two years as researchers track how many new cases of Alzheimer’s disease emerge.
A total of 7,200 individuals, 3,600 from Indiana and 3,600 from Florida, will participate in the trials. Each participant is 65 years old or older, has had at least one visit to a primary care practice within the year prior, and can communicate in English or Spanish.
Considering that the estimated prevalence of dementia in primary care in the US is 6 percent of patients, but the percentage of recognized cases is 2 percent, researchers believe that the passive digital marker tool will be able to help raise the number of dementia cases identified significantly, the press release noted.
AI is increasingly being applied in clinical care. In April, researchers from the RIKEN Center for Advanced Intelligence Project found that heart disease diagnoses became more accurate following the implementation of an AI model into ultrasounds.
Initially, a group of researchers created an AI model that could define a healthy fetal heart. They then exposed the model to thousands of ultrasounds and defined each as either healthy or unhealthy. Following another round of deep learning, researchers tested the tool by providing diagnoses with and without the AI model. They found that the AI-based decision charts led to more accurate diagnoses.