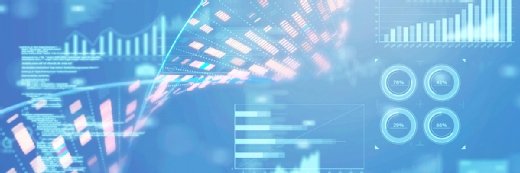
Yuichiro Chino/Moment via Getty
Researchers Develop ML Tool for Studying Characteristics of Single Cells
Researchers have developed new machine-learning software that may help pinpoint the exact causes of genetic-based diseases by enabling analyses of single-cell characteristics.
Researchers from the Children’s Hospital of Philadelphia (CHOP) and the New Jersey Institute of Technology (NJIT) developed new machine-learning (ML) software to analyze multiple characteristics of a single cell, which may lead to insights into the causes of genetic disease.
The press release states that the tool enables researchers to perform single-cell sequencing, which helps them see how one change in a cell can lead to several others and how specific aspects of a cell impact its interaction within its microenvironment. Using single-cell sequencing, researchers can study gene expression, messenger RNA, proteins, and organelles within the cells in much greater detail than previously possible.
This type of sequencing is particularly relevant for cancer research because it can be leveraged to evaluate the effects of a mutation that may only affect a small number of cells, the press release notes. However, challenges can arise when studying a single cell’s characteristics. Because each characteristic is studied individually, its connections with other characteristics, like how a genetic variant may impact messenger RNA, may not be clear, even when comparing data from the same cell.
To address this, the research team developed an ML tool known as single-cell multimodal deep clustering (scMDC) to analyze data on the different characteristics of a cell. From there, they conducted simulated and real-data experiments to evaluate the tool’s performance, a process they outlined in a study published last week in Nature Communications.
Overall, the researchers found that scMDC outperformed existing single-cell single-modal and multimodal clustering methods on single-cell multimodal data sets. These findings highlight the tool’s potential for profiling what is happening within a single cell across multiple biological processes simultaneously and better characterizing relationships between changes in that cell, according to the press release.
“With this tool, we can better understand a single cell as an entity and not just as a fragmented unit,” said Hakon Hakonarson, MD, PhD, director of the Center for Applied Genomics at CHOP and a senior author of the study, in the press release. “This is a significant advancement and allows us to integrate and put all of this information into biological perspective, which is particularly important when considering information on different diseases.”
This study is part of ongoing research on leveraging AI to help combat genetic disease.
In August, researchers from the University of Texas Southwestern Medical Center shared that they had developed AI algorithms capable of predicting the role and structure of DNA’s regulatory elements. This has the potential to lead to an understanding of how genetic mutations cause disease and how scientists may be able to address these mutations.
To investigate how DNA sequence influences the function of these regulatory elements, the research team developed a deep-learning (DL) model known as Sei. The model sorts sections of noncoding DNA into 40 sequence classes, categorizing each snippet of DNA by 'job,' such as enhancer for stem cells.
When applied to human genomic data, the tool could characterize the regulatory architecture of 47 traits and diseases, enabling the researchers to gain insights into how mutations in these elements cause certain pathologies.
The research team then created a second AI tool, known as Orca, which could predict the 3D architecture of DNA in chromosomes based on the sequence. Using Orca, the researchers were able to generate new hypotheses about how DNA sequences may control its 3D structure. The research team plans to use Sei and Orca to further investigate the role of genetic mutations in causing diseases, which may lead to new treatment approaches.