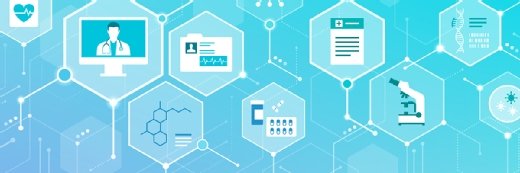
elenabs/istock via getty images
Deep-Learning Model Identifies Lung Cancer Risk Using Chest X-rays
A deep-learning model may help identify individuals who could benefit from lung cancer screening, including those missed by Medicare screening eligibility criteria.
In a recent study, researchers validated a deep-learning (DL) model to predict lung cancer risk using chest radiographs and EMR data.
The Centers for Disease Control and Prevention (CDC) report that lung cancer is the third most common cancer in the US, with more people dying from lung cancer than any other type. According to the study, lung cancer screening with chest computed tomography (CT) reduces lung cancer mortality by 20 to 24 percent, but less than 5 percent of eligible Americans are screened.
The researchers explained that this lack of screening uptake might be partly due to limitations in US Centers for Medicare & Medicaid Services (CMS) guidelines. In 2015, CMS established coverage for lung cancer screening for individuals aged 55 to 77 years with a smoking history of 30 pack-years or more who are currently smoking cigarettes or quit within the past 15 years. Pack years are defined as packs of cigarettes smoked per day multiplied by years of smoking.
However, the authors stated that over 50 percent of lung cancers occur in those ineligible for screening per CMS criteria, which may perpetuate disparities by excluding female patients or members of minoritized racial groups. Many of these patients are at high risk for lung cancer despite less cigarette smoking exposure and younger age, the research team explained.
Last year, CMS adopted updated lung cancer screening eligibility guidelines based on the 2021 US Preventive Services Task Force (USPSTF) recommendation to expand eligibility to include patients as young as 50 and lower the pack-year threshold from 30 or more to 20. This effort expanded eligibility but did not address poor participation in lung cancer screening, according to the researchers.
They stated that low uptake could be a result of difficulty remembering eligibility criteria, lack of time to obtain a smoking history, and absence of EMR reminders. To address this, the research team sought to validate an automated lung cancer risk tool to complement CMS criteria.
The tool, a DL-based model known as CXR-LC, is designed to identify high-risk individuals who smoke cigarettes for lung cancer screening CT based on readily available EMR data: age, sex, current cigarette smoking status, and a chest radiograph image.
To validate CXR-LC, developed and tested in previous research, the researchers conducted a prognostic study comparing CXR-LC risk estimates with CMS screening guidelines using patient data from 14,737 patients in the Mass General Brigham health system. Participants were patients who currently or formerly smoked cigarettes with an outpatient posterior-anterior chest radiograph between Jan. 1, 2013, and Dec. 31, 2014, with no history of lung cancer or screening CT. The main outcome measured was the six-year incidence of lung cancer.
Overall, the study cohort had a 2.4 percent rate of incident lung cancer over six years. CMS eligibility could be determined for 42.6 percent of patients using smoking pack-year and quit-dates found in the EMR. Among those eligible by both CXR-LC and 2022 CMS criteria, lung cancer rates were higher at 8.5 percent compared to 2.8 percent among patients eligible by CMS criteria alone.
The researchers also found that patients eligible by CXR-LC but not 2022 CMS criteria had a high six-year incidence of lung cancer at 3.3 percent. In the 57.4 percent of the cohort for which CMS eligibility was unknown, CXR-LC eligible patients had a five-fold higher rate of lung cancer than ineligible patients — 2.5 percent versus 0.5 percent.
These findings showed that CXR-LC accurately identified patients at high risk for lung cancer, including those missed by Medicare lung cancer screening eligibility criteria, which suggests that the model can help identify patients who may benefit from CT-based lung cancer screening, the authors concluded.