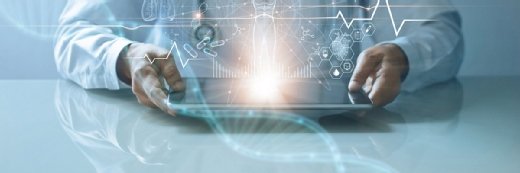
Getty Images/iStockphoto
Machine-Learning Model Estimates Risk of Adverse Prescription Opioid Outcomes
A machine-learning model trained on administrative data can estimate adverse outcome risk within 30 days of opioid dispensation.
A study published last week in JAMA Network Open found that a machine learning (ML)-based model leveraging available administrative data can accurately estimate adverse opioid outcomes, which could help enhance opioid stewardship and prescription drug monitoring programs (PDMPs).
The opioid epidemic has created a major population health burden internationally, with a significant portion of opioid-related adverse events stemming from prescription opioids. The Centers for Disease Control and Prevention (CDC) indicate that from 1999 to 2020, over 263,000 people died from overdoses involving prescription opioids in the US, with overdose deaths involving prescription opioids increasing by nearly five times in that decade.
The study noted that Canada faces similar issues with prescription opioid use, with research suggesting that the country has some of the highest rates of opioid prescribing in the world, which plays a significant role in driving its opioid crisis.
Many efforts to prevent opioid-related adverse outcomes incorporate opioid stewardship and PDMPs. Some public and population health stakeholders have shown interest in integrating ML-based risk prediction to support prescription opioid stewardship approaches, but the authors stated that they were not aware of any health jurisdictions in Canada that use ML approaches in their opioid stewardship programs at the time of the study’s publication.
To show the potential value of incorporating ML in opioid stewardship efforts, the researchers developed and validated a proof-of-concept XGBoost ML model for use by the College of Physicians and Surgeons of Alberta (CPSA) that could estimate the risk of adverse outcomes within 30 days of an opioid dispensation in the province of Alberta, Canada.
According to the study, the CPSA plays a major role in prescription opioid stewardship, overseeing Tracked Prescription Program (TPP) Alberta, a province-based PDMP. To demonstrate an ML model’s potential utility, the researchers built and trained their model using only prescription drug records aligned with the type of local, population-level data to which TPP Alberta would have access.
The model leveraged administrative data from 853,324 adults in Alberta who received at least one opioid dispensation from a community pharmacy within the province, which represented 145,016 reported outcome events and 6.1 million opioid dispensations from Jan. 1, 2018, to Dec. 31, 2019.
Using these data, opioid-related adverse outcomes were identified as hospitalization, an emergency department visit, or mortality within 30 days of an opioid dispensation. The model was developed on 2018 data to estimate the risk of these outcomes. The 2019 data was used for validation to evaluate model performance.
Of the outcome events, 77,326 occurred within 30 days of a dispensation in the validation set.
The top 0.1 percentile of estimated risk had a positive likelihood ratio (LR) of 28.7. LR, a statistical metric used to summarize diagnostic accuracy, describes how much more or less likely patients with the disease or outcome are to have a particular result than patients without the disease or outcome.
LR is also related to pre-and post-test probabilities, which capture the odds of a patient having a particular disease or outcome as estimated both before and after the test result is known, according to the American Society for Microbiology. These probabilities can help researchers or clinicians take a test’s performance into account and interpret test results.
The LR in this study translated to a post-test probability of 43.1 percent compared to a pre-test probability of 3 percent.
These findings suggest that ML models have the potential to help PDMPs identify and intervene in cases of high-risk opioid prescribing to fulfill opioid stewardship mandates if given comprehensive access to routinely collected administrative health data, the researchers stated.