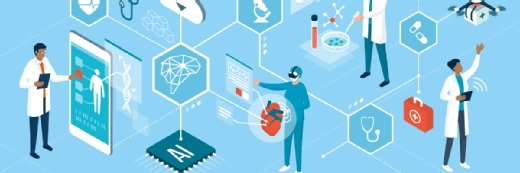
Getty Images
New Machine-Learning Model Identifies Long COVID Subtypes
A newly developed machine-learning tool uses EHR data to find common symptoms among people with long COVID and identify condition subtypes.
Researchers from the University of California, Berkeley have developed machine-learning (ML) software that uses entries in EHRs to shed light on long COVID, including finding common symptoms and identifying subtypes of the condition.
According to data collected from June 1 to June 13, 2022, by the US Census Bureau and analyzed by the Centers for Disease Control and Prevention’s (CDC) National Center for Health Statistics (NCHS), over 40 percent of US adults reported having COVID-19 in the past. Of these, 19 percent are still experiencing COVID-19 symptoms, a condition known as long COVID.
Overall, 7.5 percent of US adults have long COVID, defined by the CDC as symptoms lasting three or more months after first contracting the virus that they did not have prior to their COVID-19 infection. However, much is still unknown about the condition and its symptoms. The CDC reports that symptoms can vary widely and include neurological, respiratory, heart, digestive, and other symptoms. These may last for weeks, months, or years.
These gaps in knowledge about long COVID have spurred many research efforts, including those leveraging artificial intelligence and ML. In a recent study published in eBioMedicine, a team led by researchers from the Lawrence Berkeley National Laboratory (Berkeley Lab) showcased the development of an ML model designed to glean insights into long COVID using EHR data and support precision clinical management strategies.
The model works by computationally modeling post-acute sequelae of SARS CoV-2 infection (PASC) phenotype data sourced from EHR analysis and assessing phenotypic similarity between patients using semantic similarity, a bioinformatics metric used to compare different types of biomedical entities based on their biological role, rather than what they look like.
The researchers developed and validated the tool using data from 6,469 patients diagnosed with long COVID following confirmed COVID-19 infections.
“Basically, we found long COVID features in the EHR data for each long COVID patient, and then assessed patient-patient similarity using semantic similarity, which essentially allows ‘fuzzy matching’ between features – for example, ‘cough’ is not the same as ‘shortness of breath,’ but they are similar since they both involve lung problems,” explained Justin Reese, PhD, a computer research scientist in Berkeley Lab’s Biosciences Area, in the press release. “We compare all symptoms for the pair of the patients in this way, and get a score of how similar the two long COVID patients are. We can then perform unsupervised machine learning on these scores to find different subtypes of long COVID.”
The team leveraged ML to cluster patients into groups based on patient-patient similarity scores. These groups were then characterized via an analysis of relationships between symptoms and pre-existing diseases and other demographic features, like race, age, and gender.
Overall, the researchers found six clusters of PASC patients, each with distinct profiles of phenotypic abnormalities. These included clusters with distinct neuropsychiatric, pulmonary, and cardiovascular abnormalities. The team also identified a cluster associated with broad, severe manifestations and increased mortality. Cluster membership was associated with a range of pre-existing conditions and measures of disease severity.
The researchers concluded that the tool could provide a foundation for patient subgroup stratification, which may advance further research and precision clinical management strategies.