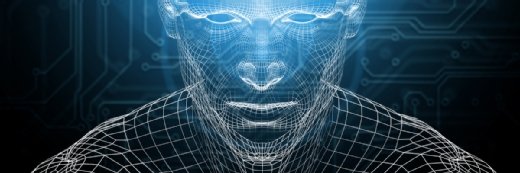
Getty Images
Machine Learning Tools Help Predict Alzheimer's Progression
Researchers from Weill Cornell Medicine have used machine learning tools to identify the most accurate timelines and means of anticipating cognitive decline.
A new study published in PLOS ONE leveraged machine learning (ML) models to identify the most accurate means and timelines for anticipating the advancement of Alzheimer’s disease in people who are either cognitively normal or experiencing mild cognitive impairment.
Alzheimer's can take years or decades to progress before a patient begins to exhibit symptoms and some rates of cognitive decline vary significantly from one individual to another, the press release states. Therefore, forecasting the rate of disease advancement can be a challenge for clinicians.
“When we can confidently say someone has dementia, it is too late. A lot of damage has already happened to the brain, and it’s irreversible damage,” said senior author Mert Sabuncu, PhD, associate professor of electrical and computer engineering in the College of Engineering and of electrical engineering in radiology at Weill Cornell Medicine, in the press release.
“We really need to be able to catch Alzheimer’s disease early on,” Sabuncu continued, “and be able to tell who’s going to progress fast and who’s going to progress slower, so that we can stratify the different risk groups and be able to deploy whatever treatment options we have.”
To do this, the research team turned to machine learning to analyze the wealth of biomedical data generated by patients throughout their care journeys to see if these data could help improve Alzheimer's advancement forecasting.
“What we were really interested in is, can we look at these data and tell whether a person will progress in upcoming years?” Sabuncu said. “And importantly, can we do a better job in forecasting when we combine all the follow-up datapoints we have on individual subjects?”
The researchers gathered five years’ worth of data from 1,404 patients who were either cognitively normal or had mild cognitive impairment. These data were sourced from the Alzheimer’s Disease Neuroimaging Initiative, and captured various datapoints, such as an individual’s genetic history, baseline diagnosis, PET and MRI scans, and biomarker values.
Overall, the research team uncovered several insights into how to more accurately predict cognitive decline.
The study’s findings indicate that predicting the future decline into dementia for individuals with mild cognitive impairment is easier and more accurate than it is for cognitively normal, or asymptomatic, individuals.
The researchers also found that the predictions for cognitively normal subjects are less accurate for longer time horizons, but the opposite is true for individuals with mild cognitive impairment.
In terms of the means used to predict decline, the ML models showed that MRI is a useful prognostic tool for people in both stages. In particular, MRI data were found most informative for asymptomatic cases and are particularly helpful for predicting if someone’s going to develop symptoms over the next three years, but less helpful for forecasting for people with mild cognitive impairment.
Conversely, tools that track molecular biomarkers, such as PET scans, appear to be more effective for people experiencing mild cognitive impairment.
The ML modeling also revealed that predicting a person will move from being asymptomatic to exhibiting mild symptoms is much easier for a time horizon of one year than of five years. Predicting if someone will decline from mild cognitive impairment into Alzheimer’s, though, is most accurate on a longer timeline of about four years.
Moving forward, the researchers plan to modify the ML models so that they can process complete imaging or genomic data, rather than just summary measurements, which will help gather more complete information and potentially boost predictive accuracy.