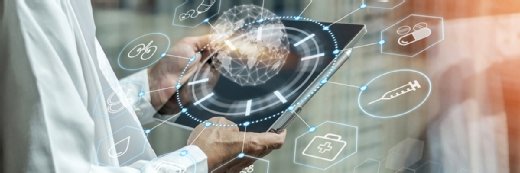
Chinnapong - stock.adobe.com
Deep Learning Predicts Heart Disease, Stroke Death Risk Using Single X-ray
Researchers have developed a deep-learning model that uses a single chest X-ray to predict the 10-year risk of death from a heart attack or stroke.
Researchers from Massachusetts General Hospital and Brigham and Women's Hospital have developed a deep-learning model that uses a single chest X-ray to predict the 10-year risk of death from a heart attack or stroke stemming from atherosclerotic cardiovascular disease, according to research presented this week at the annual meeting of the Radiological Society of North America (RSNA).
Cardiovascular disease risk is a major population health and chronic disease concern. The press release states that current guidelines recommend estimating the 10-year risk of major adverse cardiovascular disease events to establish who should get a statin for primary prevention.
Risk is calculated using the atherosclerotic cardiovascular disease (ASCVD) risk score, a statistical model that considers multiple patient variables, such as age, sex, race, systolic blood pressure, hypertension treatment, smoking, type 2 diabetes, and blood tests. For patients with a 10-year risk of 7.5 percent or higher, statin medication is recommended.
However, calculating risk in this way can be a challenge.
"The variables necessary to calculate ASCVD risk are often not available, which makes approaches for population-based screening desirable," said the study's lead author, Jakob Weiss, MD, a radiologist affiliated with the Cardiovascular Imaging Research Center at Massachusetts General Hospital and the AI in Medicine program at the Brigham and Women's Hospital in Boston, in the press release. "As chest X-rays are commonly available, our approach may help identify individuals at high risk."
The researchers’ approach involves using a deep-learning (DL) model to analyze X-ray images, which can give clinicians additional insight into cardiovascular risk.
"We've long recognized that X-rays capture information beyond traditional diagnostic findings, but we haven't used this data because we haven't had robust, reliable methods," Weiss said. "Advances in AI are making it possible now."
To utilize X-ray data to evaluate cardiovascular disease risk, the research team trained a DL model, known as CXR-CVD risk, using single patient chest X-ray inputs to predict cardiovascular disease death risk. The researchers trained the model using 147,497 chest X-rays from 40,643 participants in the National Cancer Institute’s Prostate, Lung, Colorectal, and Ovarian Cancer Screening Trial, a multi-center, randomized controlled trial.
The research team tested the model on a second, independent cohort of 11,430 outpatients who had a routine outpatient chest X-ray at Mass General Brigham and were potentially eligible for statin therapy. Of these patients, 9.6 percent suffered a major adverse cardiac event over the median follow-up of 10.3 years.
Overall, the researchers found a significant association between the risk predicted by the CXR-CVD risk deep-learning model and observed major cardiac events. They also determined that the CXR-CVD risk model performed similarly to the established clinical standard.
"The beauty of this approach is you only need an X-ray, which is acquired millions of times a day across the world," Weiss stated. "Based on a single existing chest X-ray image, our deep learning model predicts future major adverse cardiovascular events with similar performance and incremental value to the established clinical standard."
The researchers concluded that though additional research is needed to validate the model, these findings indicate its potential utility as a clinical decision-support tool.
This research is the latest effort to leverage AI to predict cardiovascular risk.
In April, Johns Hopkins researchers created an AI system called Survival Study of Cardiac Arrhythmia Risk (SSCAR), designed to detect patterns in cardiac imaging and patient demographic data to predict the probability of sudden cardiac arrest in the following 10 years.
They also developed a neural network that used 10 years of patient data, including 22 patient factors, such as age, weight, and race, to generate predictions. Patient data from 60 US health centers were used to validate the two algorithms.